Planet Python
Last update: June 06, 2025 01:42 AM UTC
June 05, 2025
Mike Driscoll
Parsing XML Comments with Python
The Extensible Markup Language (XML) is a markup format originally for XML. Some companies use XML as a data serialization format or for configuration. Recently, I needed to learn how to uncomment some lines in an XML file to enable some settings that were defined in the XML.
Fortunately, Python’s xml
module provides a way to do this. Let’s find out how!
AI Answers Might Not Work
When I used Google to search for an answer to this question: “How to edit comments in XML with Python”, Google Gemini piped up with this answer:
import xml.etree.ElementTree as ET xml_file = "PATH_TO_XML" tree = ET.parse(xml_file) root = tree.getroot() for element in root.iter(): if isinstance(element.tag, str) and element.tag.startswith('{'): continue if element.tag == ET.Comment: text = element.text.strip() print(text)
Unfortunately, this code does not work. But it is close.
If you look through StackOverflow and similar forums, you will discover that you need a custom parser. Here’s how to create one:
import xml.etree.ElementTree as ET xml_file = r"PATH_TO_XML" parser = ET.XMLParser(target=ET.TreeBuilder(insert_comments=True)) tree = ET.parse(xml_file, parser) root = tree.getroot() for element in root.iter(): if isinstance(element.tag, str) and element.tag.startswith('{'): continue if element.tag == ET.Comment: text = element.text.strip() print(text)
The key point here is to create an instance of ET.XMLParser and set insert_comments to True. Then the code will work.
Note that this example just prints out the commented text. You would need to do something like this to grab the commented text and reinsert it as a valid XML element:
for element in root.iter(): if isinstance(element.tag, str) and element.tag.startswith('{'): continue if element.tag == ET.Comment: text = element.text.strip() if "COMMENTED CODE SUBSTRING" in text: new_element = ET.fromstring(f"<{text}>") # Insert the uncommented text as a new XML element root.insert(list(root).index(element), new_element) # Remove the element that was commented out originally root.remove(element) # Make indentation work for the output ET.indent(tree, space="\t", level=0) with open(XML_PATH, "wb") as f: tree.write(f)
Here, you loop over each element or tag in the XML. You check if the element is a comment type. If it is, you check for the substring you are looking for in the comment’s text. When you find the substring, you extract the entire string from the comment, create a new element, insert it as a regular element, and remove the comment.
Wrapping Up
XML is a handy format, and Python includes several different methods of working with XML in its xml
module. Several different third-party XML modules, such as lxml, are also great alternatives. If you work with XML, hopefully you will find this article helpful.
Have fun and happy coding!
The post Parsing XML Comments with Python appeared first on Mouse Vs Python.
Glyph Lefkowitz
I Think I’m Done Thinking About genAI For Now
The Problem
Like many other self-styled thinky programmer guys, I like to imagine myself as a sort of Holmesian genius, making trenchant observations, collecting them, and then synergizing them into brilliant deductions with the keen application of my powerful mind.
However, several years ago, I had an epiphany in my self-concept. I finally understood that, to the extent that I am usefully clever, it is less in a Holmesian idiom, and more, shall we say, Monkesque.
For those unfamiliar with either of the respective franchises:
- Holmes is a towering intellect honed by years of training, who catalogues intentional, systematic observations and deduces logical, factual conclusions from those observations.
- Monk, on the other hand, while also a reasonably intelligent guy, is highly neurotic, wracked by unresolved trauma and profound grief. As both a consulting job and a coping mechanism, he makes a habit of erratically wandering into crime scenes, and, driven by a carefully managed jenga tower of mental illnesses, leverages his dual inabilities to solve crimes. First, he is unable to filter out apparently inconsequential details, building up a mental rat’s nest of trivia about the problem; second, he is unable to let go of any minor incongruity, obsessively ruminating on the collection of facts until they all make sense in a consistent timeline.
Perhaps surprisingly, this tendency serves both this fictional wretch of a detective, and myself, reasonably well. I find annoying incongruities in abstractions and I fidget and fiddle with them until I end up building something that a lot of people like, or perhaps something that a smaller number of people get really excited about. At worst, at least I eventually understand what’s going on. This is a self-soothing activity but it turns out that, managed properly, it can very effectively soothe others as well.
All that brings us to today’s topic, which is an incongruity I cannot smooth out or fit into a logical framework to make sense. I am, somewhat reluctantly, a genAI skeptic. However, I am, even more reluctantly, exposed to genAI Discourse every damn minute of every damn day. It is relentless, inescapable, and exhausting.
This preamble about personality should hopefully help you, dear reader, to understand how I usually address problematical ideas by thinking and thinking and fidgeting with them until I manage to write some words — or perhaps a new open source package — that logically orders the ideas around it in a way which allows my brain to calm down and let it go, and how that process is important to me.
In this particular instance, however, genAI has defeated me. I cannot make it make sense, but I need to stop thinking about it anyway. It is too much and I need to give up.
My goal with this post is not to convince anyone of anything in particular — and we’ll get to why that is a bit later — but rather:
- to set out my current understanding in one place, including all the various negative feelings which are still bothering me, so I can stop repeating it elsewhere,
- to explain why I cannot build a case that I think should be particularly convincing to anyone else, particularly to someone who actively disagrees with me,
- in so doing, to illustrate why I think the discourse is so fractious and unresolvable, and finally
- to give myself, and hopefully by proxy to give others in the same situation, permission to just peace out of this nightmare quagmire corner of the noosphere.
But first, just because I can’t prove that my interlocutors are Wrong On The Internet, doesn’t mean I won’t explain why I feel like they are wrong.
The Anti-Antis
Most recently, at time of writing, there have been a spate of “the genAI discourse is bad” articles, almost exclusively written from the perspective of, not boosters exactly, but pragmatically minded (albeit concerned) genAI users, wishing for the skeptics to be more pointed and accurate in our critiques. This is anti-anti-genAI content.
I am not going to link to any of these, because, as part of their self-fulfilling prophecy about the “genAI discourse”, they’re also all bad.
Mostly, however, they had very little worthwhile to respond to because they were straw-manning their erstwhile interlocutors. They are all getting annoyed at “bad genAI criticism” while failing to engage with — and often failing to even mention — most of the actual substance of any serious genAI criticism. At least, any of the criticism that I’ve personally read.
I understand wanting to avoid a callout or Gish-gallop culture and just express your own ideas. So, I understand that they didn’t link directly to particular sources or go point-by-point on anyone else’s writing. Obviously I get it, since that’s exactly what this post is doing too.
But if you’re going to talk about how bad the genAI conversation is, without even mentioning huge categories of problem like “climate impact” or “disinformation”1 even once, I honestly don’t know what conversation you’re even talking about. This is peak “make up a guy to get mad at” behavior, which is especially confusing in this circumstance, because there’s an absolutely huge crowd of actual people that you could already be mad at.
The people writing these pieces have historically seemed very thoughtful to me. Some of them I know personally. It is worrying to me that their critical thinking skills appear to have substantially degraded specifically after spending a bunch of time intensely using this technology which I believe has a scary risk of degrading one’s critical thinking skills. Correlation is not causation or whatever, and sure, from a rhetorical perspective this is “post hoc ergo propter hoc” and maybe a little “ad hominem” for good measure, but correlation can still be concerning.
Yet, I cannot effectively respond to these folks, because they are making a practical argument that I cannot, despite my best efforts, find compelling evidence to refute categorically. My experiences of genAI are all extremely bad, but that is barely even anecdata. Their experiences are neutral-to-positive. Little scientific data exists. How to resolve this?2
The Aesthetics
As I begin to state my own position, let me lead with this: my factual analysis of genAI is hopelessly negatively biased. I find the vast majority of the aesthetic properties of genAI to be intensely unpleasant.
I have been trying very hard to correct for this bias, to try to pay attention to the facts and to have a clear-eyed view of these systems’ capabilities. But the feelings are visceral, and the effort to compensate is tiring. It is, in fact, the desire to stop making this particular kind of effort that has me writing up this piece and trying to take an intentional break from the subject, despite its intense relevance.
When I say its “aesthetic qualities” are unpleasant, I don’t just mean the aesthetic elements of output of genAIs themselves. The aesthetic quality of genAI writing, visual design, animation and so on, while mostly atrocious, is also highly variable. There are cherry-picked examples which look… fine. Maybe even good. For years now, there have been, famously, literally award-winning aesthetic outputs of genAI3.
While I am ideologically predisposed to see any “good” genAI art as accruing the benefits of either a survivorship bias from thousands of terrible outputs or simple plagiarism rather than its own inherent quality, I cannot deny that in many cases it is “good”.
However, I am not just talking about the product, but the process; the aesthetic experience of interfacing with the genAI system itself, rather than the aesthetic experience of the outputs of that system.
I am not a visual artist and I am not really a writer4, particularly not a writer of fiction or anything else whose experience is primarily aesthetic. So I will speak directly to the experience of software development.
I have seen very few successful examples of using genAI to produce whole, working systems. There are no shortage of highly public miserable failures, particularly from the vendors of these systems themselves, where the outputs are confused, self-contradictory, full of subtle errors and generally unusable. While few studies exist, it sure looks like this is an automated way of producing a Net Negative Productivity Programmer, throwing out chaff to slow down the rest of the team.5
Juxtapose this with my aforementioned psychological motivations, to wit, I want to have everything in the computer be orderly and make sense, I’m sure most of you would have no trouble imagining that sitting through this sort of practice would make me extremely unhappy.
Despite this plethora of negative experiences, executives are aggressively mandating the use of AI6. It looks like without such mandates, most people will not bother to use such tools, so the executives will need muscular policies to enforce its use.7
Being forced to sit and argue with a robot while it struggles and fails to produce a working output, while you have to rewrite the code at the end anyway, is incredibly demoralizing. This is the kind of activity that activates every single major cause of burnout at once.
But, at least in that scenario, the thing ultimately doesn’t work, so there’s a hope that after a very stressful six month pilot program, you can go to management with a pile of meticulously collected evidence, and shut the whole thing down.
I am inclined to believe that, in fact, it doesn’t work well enough to be used this way, and that we are going to see a big crash. But that is not the most aesthetically distressing thing. The most distressing thing is that maybe it does work; if not well enough to actually do the work, at least ambiguously enough to fool the executives long-term.
This project, in particular, stood out to me as an example. Its author, a self-professed “AI skeptic” who “thought LLMs were glorified Markov chain generators that didn’t actually understand code and couldn’t produce anything novel”, did a green-field project to test this hypothesis.
Now, this particular project is not totally inconsistent with a world in which LLMs cannot produce anything novel. One could imagine that, out in the world of open source, perhaps there is enough “OAuth provider written in TypeScript” blended up into the slurry of “borrowed8” training data that the minor constraint of “make it work on Cloudflare Workers” is a small tweak9. It is not fully dispositive of the question of the viability of “genAI coding”.
But it is a data point related to that question, and thus it did make me contend with what might happen if it were actually a fully demonstrative example. I reviewed the commit history, as the author suggested. For the sake of argument, I tried to ask myself if I would like working this way. Just for clarity on this question, I wanted to suspend judgement about everything else; assuming:
- the model could be created with ethically, legally, voluntarily sourced training data
- its usage involved consent from labor rather than authoritarian mandates
- sensible levels of energy expenditure, with minimal CO2 impact
- it is substantially more efficient to work this way than to just write the code yourself
and so on, and so on… would I like to use this magic robot that could mostly just emit working code for me? Would I use it if it were free, in all senses of the word?
No. I absolutely would not.
I found the experience of reading this commit history and imagining myself using such a tool — without exaggeration — nauseating.
Unlike many programmers, I love code review. I find that it is one of the best parts of the process of programming. I can help people learn, and develop their skills, and learn from them, and appreciate the decisions they made, develop an impression of a fellow programmer’s style. It’s a great way to build a mutual theory of mind.
Of course, it can still be really annoying; people make mistakes, often can’t see things I find obvious, and in particular when you’re reviewing a lot of code from a lot of different people, you often end up having to repeat explanations of the same mistakes. So I can see why many programmers, particularly those more introverted than I am, hate it.
But, ultimately, when I review their code and work hard to provide clear and actionable feedback, people learn and grow and it’s worth that investment in inconvenience.
The process of coding with an “agentic” LLM appears to be the process of carefully distilling all the worst parts of code review, and removing and discarding all of its benefits.
The lazy, dumb, lying robot asshole keeps making the same mistakes over and over again, never improving, never genuinely reacting, always obsequiously pretending to take your feedback on board.
Even when it “does” actually “understand” and manages to load your instructions into its context window, 200K tokens later it will slide cleanly out of its memory and you will have to say it again.
All the while, it is attempting to trick you. It gets most things right, but it consistently makes mistakes in the places that you are least likely to notice. In places where a person wouldn’t make a mistake. Your brain keeps trying to develop a theory of mind to predict its behavior but there’s no mind there, so it always behaves infuriatingly randomly.
I don’t think I am the only one who feels this way.
The Affordances
Whatever our environments afford, we tend to do more of. Whatever they resist, we tend to do less of. So in a world where we were all writing all of our code and emails and blog posts and texts to each other with LLMs, what do they afford that existing tools do not?
As a weirdo who enjoys code review, I also enjoy process engineering. The central question of almost all process engineering is to continuously ask: how shall we shape our tools, to better shape ourselves?
LLMs are an affordance for producing more text, faster. How is that going to shape us?
Again arguing in the alternative here, assuming the text is free from errors and hallucinations and whatever, it’s all correct and fit for purpose, that means it reduces the pain of circumstances where you have to repeat yourself. Less pain! Sounds great; I don’t like pain.
Every codebase has places where you need boilerplate. Every organization has defects in its information architecture that require repetition of certain information rather than a link back to the authoritative source of truth. Often, these problems persist for a very long time, because it is difficult to overcome the institutional inertia required to make real progress rather than going along with the status quo. But this is often where the highest-value projects can be found. Where there’s muck, there’s brass.
The process-engineering function of an LLM, therefore, is to prevent fundamental problems from ever getting fixed, to reward the rapid-fire overwhelm of infrastructure teams with an immediate, catastrophic cascade of legacy code that is now much harder to delete than it is to write.
There is a scene in Game of Thrones where Khal Drogo kills himself. He does so by replacing a stinging, burning, therapeutic antiseptic wound dressing with some cool, soothing mud. The mud felt nice, addressed the immediate pain, removed the discomfort of the antiseptic, and immediately gave him a lethal infection.
The pleasing feeling of immediate progress when one prompts an LLM to solve some problem feels like cool mud on my brain.
The Economics
We are in the middle of a mania around this technology. As I have written about before, I believe the mania will end. There will then be a crash, and a “winter”. But, as I may not have stressed sufficiently, this crash will be the biggest of its kind — so big, that it is arguably not of a kind at all. The level of investment in these technologies is bananas and the possibility that the investors will recoup their investment seems close to zero. Meanwhile, that cost keeps going up, and up, and up.
Others have reported on this in detail10, and I will not reiterate that all here, but in addition to being a looming and scary industry-wide (if we are lucky; more likely it’s probably “world-wide”) economic threat, it is also going to drive some panicked behavior from management.
Panicky behavior from management stressed that their idea is not panning out is, famously, the cause of much human misery. I expect that even in the “good” scenario, where some profit is ultimately achieved, will still involve mass layoffs rocking the industry, panicked re-hiring, destruction of large amounts of wealth.
It feels bad to think about this.
The Energy Usage
For a long time I believed that the energy impact was overstated. I am even on record, about a year ago, saying I didn’t think the energy usage was a big deal. I think I was wrong about that.
It initially seemed like it was letting regular old data centers off the hook. But recently I have learned that, while the numbers are incomplete because the vendors aren’t sharing information, they’re also extremely bad.11
I think there’s probably a version of this technology that isn’t a climate emergency nightmare, but that’s not the version that the general public has access to today.
The Educational Impact
LLMs are making academic cheating incredibly rampant.12
Not only is it so common as to be nearly universal, it’s also extremely harmful to learning.13
For learning, genAI is a forklift at the gym.
To some extent, LLMs are simply revealing a structural rot within education and academia that has been building for decades if not centuries. But it was within those inefficiencies and the inconveniences of the academic experience that real learning was, against all odds, still happening in schools.
LLMs produce a frictionless, streamlined process where students can effortlessly glide through the entire credential, learning nothing. Once again, they dull the pain without regard to its cause.
This is not good.
The Invasion of Privacy
This is obviously only a problem with the big cloud models, but then, the big cloud models are the only ones that people actually use. If you are having conversations about anything private with ChatGPT, you are sending all of that private information directly to Sam Altman, to do with as he wishes.
Even if you don’t think he is a particularly bad guy, maybe he won’t even create the privacy nightmare on purpose. Maybe he will be forced to do so as a result of some bizarre kafkaesque accident.14
Imagine the scenario, for example, where a woman is tracking her cycle and uploading the logs to ChatGPT so she can chat with it about a health concern. Except, surprise, you don’t have to imagine, you can just search for it, as I have personally, organically, seen three separate women on YouTube, at least one of whom lives in Texas, not only do this on camera but recommend doing this to their audiences.
Citation links withheld on this particular claim for hopefully obvious reasons.
I assure you that I am neither particularly interested in menstrual products nor genAI content, and if I am seeing this more than once, it is probably a distressingly large trend.
The Stealing
The training data for LLMs is stolen. I don’t mean like “pirated” in the sense where someone illicitly shares a copy they obtained legitimately; I mean their scrapers are ignoring both norms15 and laws16 to obtain copies under false pretenses, destroying other people’s infrastructure17.
The Fatigue
I have provided references to numerous articles outlining rhetorical and sometimes data-driven cases for the existence of certain properties and consequences of genAI tools. But I can’t prove any of these properties, either at a point in time or as a durable ongoing problem.
The LLMs themselves are simply too large to model with the usual kind of heuristics one would use to think about software. I’d sooner be able to predict the physics of dice in a casino than a 2 trillion parameter neural network. They resist scientific understanding, not just because of their size and complexity, but because unlike a natural phenomenon (which could of course be considerably larger and more complex) they resist experimentation.
The first form of genAI resistance to experiment is that every discussion is a
motte-and-bailey. If
I use a free model and get a bad result I’m told it’s because I should have
used the paid model. If I get a bad result with ChatGPT I should have used
Claude. If I get a bad result with a chatbot I need to start using an agentic
tool. If an agentic tool deletes my hard drive by putting os.system(“rm -rf
~/”)
into sitecustomize.py
then I guess I should have built my own MCP
integration with a completely novel heretofore never even considered security
sandbox or something?
What configuration, exactly, would let me make a categorical claim about these things? What specific methodological approach should I stick to, to get reliably adequate prompts?
For the record though, if the idea of the free models is that they are going to be provocative demonstrations of the impressive capabilities of the commercial models, and the results are consistently dogshit, I am finding it increasingly hard to care how much better the paid ones are supposed to be, especially since the “better”-ness cannot really be quantified in any meaningful way.
The motte-and-bailey doesn’t stop there though. It’s a war on all fronts. Concerned about energy usage? That’s OK, you can use a local model. Concerned about infringement? That’s okay, somewhere, somebody, maybe, has figured out how to train models consensually18. Worried about the politics of enriching the richest monsters in the world? Don’t worry, you can always download an “open source” model from Hugging Face. It doesn’t matter that many of these properties are mutually exclusive and attempting to fix one breaks two others; there’s always an answer, the field is so abuzz with so many people trying to pull in so many directions at once that it is legitimately difficult to understand what’s going on.
Even here though, I can see that characterizing everything this way is unfair to a hypothetical sort of person. If there is someone working at one of these thousands of AI companies that have been springing up like toadstools after a rain, and they really are solving one of these extremely difficult problems, how can I handwave that away? We need people working on problems, that’s like, the whole point of having an economy. And I really don’t like shitting on other people’s earnest efforts, so I try not to dismiss whole fields. Given how AI has gotten into everything, in a way that e.g. cryptocurrency never did, painting with that broad a brush inevitably ends up tarring a bunch of stuff that isn’t even really AI at all.
The second form of genAI resistance to experiment is the inherent obfuscation of productization. The models themselves are already complicated enough, but the products that are built around the models are evolving extremely rapidly. ChatGPT is not just a “model”, and with the rapid19 deployment of Model Context Protocol tools, the edges of all these things will blur even further. Every LLM is now just an enormous unbounded soup of arbitrary software doing arbitrary whatever. How could I possibly get my arms around that to understand it?
The Challenge
I have woefully little experience with these tools.
I’ve tried them out a little bit, and almost every single time the result has been a disaster that has not made me curious to push further. Yet, I keep hearing from all over the industry that I should.
To some extent, I feel like the motte-and-bailey characterization above is fair; if the technology itself can really do real software development, it ought to be able to do it in multiple modalities, and there’s nothing anyone can articulate to me about GPT-4o which puts it in a fundamentally different class than GPT-3.5.
But, also, I consistently hear that the subjective experience of using the premium versions of the tools is actually good, and the free ones are actually bad.
I keep struggling to find ways to try them “the right way”, the way that people I know and otherwise respect claim to be using them, but I haven’t managed to do so in any meaningful way yet.
I do not want to be using the cloud versions of these models with their potentially hideous energy demands; I’d like to use a local model. But there is obviously not a nicely composed way to use local models like this.
Since there are apparently zero models with ethically-sourced training data, and litigation is ongoing20 to determine the legal relationships of training data and outputs, even if I can be comfortable with some level of plagiarism on a project, I don’t feel that I can introduce the existential legal risk into other people’s infrastructure, so I would need to make a new project.
Others have differing opinions of course, including some within my dependency chain, which does worry me, but I still don’t feel like I can freely contribute further to the problem; it’s going to be bad enough to unwind any impact upstream. Even just for my own sake, I don’t want to make it worse.
This especially presents a problem because I have way too much stuff going on already. A new project is not practical.
Finally, even if I did manage to satisfy all of my quirky21 constraints, would this experiment really be worth anything? The models and tools that people are raving about are the big, expensive, harmful ones. If I proved to myself yet again that a small model with bad tools was unpleasant to use, I wouldn’t really be addressing my opponents’ views.
I’m stuck.
The Surrender
I am writing this piece to make my peace with giving up on this topic, at least for a while. While I do idly hope that some folks might find bits of it convincing, and perhaps find ways to be more mindful with their own usage of genAI tools, and consider the harm they may be causing, that’s not actually the goal. And that is not the goal because it is just so much goddamn work to prove.
Here, I must return to my philosophical hobbyhorse of sprachspiel. In this case, specifically to use it as an analytical tool, not just to understand what I am trying to say, but what the purpose for my speech is.
The concept of sprachspiel is most frequently deployed to describe the goal of the language game being played, but in game theory, that’s only half the story. Speech — particularly rigorously justified speech — has a cost, as well as a benefit. I can make shit up pretty easily, but if I want to do anything remotely like scientific or academic rigor, that cost can be astronomical. In the case of developing an abstract understanding of LLMs, the cost is just too high.
So what is my goal, then? To be king Canute, standing astride the shore of “tech”, whatever that is, commanding the LLM tide not to rise? This is a multi-trillion dollar juggernaut.
Even the rump, loser, also-ran fragment of it has the power to literally suffocate us in our homes22 if they so choose, completely insulated from any consequence. If the power curve starts there, imagine what the winners in this industry are going to be capable of, irrespective of the technology they’re building - just with the resources they have to hand. Am I going to write a blog post that can rival their propaganda apparatus? Doubtful.
Instead, I will just have to concede that maybe I’m wrong. I don’t have the skill, or the knowledge, or the energy, to demonstrate with any level of rigor that LLMs are generally, in fact, hot garbage. Intellectually, I will have to acknowledge that maybe the boosters are right. Maybe it’ll be OK.
Maybe the carbon emissions aren’t so bad. Maybe everybody is keeping them secret in ways that they don’t for other types of datacenter for perfectly legitimate reasons. Maybe the tools really can write novel and correct code, and with a little more tweaking, it won’t be so difficult to get them to do it. Maybe by the time they become a mandatory condition of access to developer tools, they won’t be miserable.
Sure, I even sincerely agree, intellectual property really has been a pretty bad idea from the beginning. Maybe it’s OK that we’ve made an exception to those rules. The rules were stupid anyway, so what does it matter if we let a few billionaires break them? Really, everybody should be able to break them (although of course, regular people can’t, because we can’t afford the lawyers to fight off the MPAA and RIAA, but that’s a problem with the legal system, not tech).
I come not to praise “AI skepticism”, but to bury it.
Maybe it really is all going to be fine. Perhaps I am simply catastrophizing; I have been known to do that from time to time. I can even sort of believe it, in my head. Still, even after writing all this out, I can’t quite manage to believe it in the pit of my stomach.
Unfortunately, that feeling is not something that you, or I, can argue with.
Acknowledgments
Thank you to my patrons. Normally, I would say, “who are supporting my writing on this blog”, but in the case of this piece, I feel more like I should apologize to them for this than to thank them; these thoughts have been preventing me from thinking more productive, useful things that I actually have relevant skill and expertise in; this felt more like a creative blockage that I just needed to expel than a deliberately written article. If you like what you’ve read here and you’d like to read more of it, well, too bad; I am sincerely determined to stop writing about this topic. But, if you’d like to read more stuff like other things I have written, or you’d like to support my various open-source endeavors, you can support my work as a sponsor!
-
And yes, disinformation is still an issue even if you’re “just” using it for coding. Even sidestepping the practical matter that technology is inherently political, validation and propagation of poor technique is a form of disinformation. ↩
-
I can’t resolve it, that’s the whole tragedy here, but I guess we have to pretend I will to maintain narrative momentum here. ↩
-
The story in Creative Bloq, or the NYT, if you must ↩
-
although it’s not for lack of trying, Jesus, look at the word count on this ↩
-
These are sometimes referred to as “10x” programmers, because they make everyone around them 10x slower. ↩
-
Douglas B. Laney at Forbes, Viral Shopify CEO Manifesto Says AI Now Mandatory For All Employees ↩
-
The National CIO Review, AI Mandates, Minimal Use: Closing the Workplace Readiness Gap ↩
-
Matt O’Brien at the AP, Reddit sues AI company Anthropic for allegedly ‘scraping’ user comments to train chatbot Claude ↩
-
Using the usual tricks to find plagiarism like searching for literal transcriptions of snippets of training data did not pull up anything when I tried, but then, that’s not how LLMs work these days, is it? If it didn’t obfuscate the plagiarism it wouldn’t be a very good plagiarism-obfuscator. ↩
-
David Gerard at Pivot to AI, “Microsoft and AI: spending billions to make millions”, Edward Zitron at Where’s Your Ed At, “The Era Of The Business Idiot”, both sobering reads ↩
-
James O’Donnell and Casey Crownhart at the MIT Technology Review, We did the math on AI’s energy footprint. Here’s the story you haven’t heard. ↩
-
Lucas Ropek at Gizmodo, AI Cheating Is So Out of Hand In America’s Schools That the Blue Books Are Coming Back ↩
-
James D. Walsh at the New York Magazine Intelligencer, Everyone Is Cheating Their Way Through College ↩
-
Ashley Belanger at Ars Technica, OpenAI slams court order to save all ChatGPT logs, including deleted chats ↩
-
Ashley Belanger at Ars Technica, AI haters build tarpits to trap and trick AI scrapers that ignore robots.txt ↩
-
Blake Brittain at Reuters, Judge in Meta case warns AI could ‘obliterate’ market for original works ↩
-
Xkeeper, TCRF has been getting DDoSed ↩
-
Kate Knibbs at Wired, Here’s Proof You Can Train an AI Model Without Slurping Copyrighted Content ↩
-
and, I should note, extremely irresponsible ↩
-
Porter Anderson at Publishing Perspectives, Meta AI Lawsuit: US Publishers File Amicus Brief ↩
-
It feels bizarre to characterize what feel like baseline ethical concerns this way, but the fact remains that within the “genAI community”, this places me into a tiny and obscure minority. ↩
-
Ariel Wittenberg for Politico, ‘How come I can’t breathe?’: Musk’s data company draws a backlash in Memphis ↩
Wingware
Wing Python IDE Version 11 - June 5, 2025
Wing Python IDE version 11 is now available. It improves the AI assisted development UI and adds support for Claude, Grok, Gemini, OpenAI, Perplexity, Mistral, Deepseek, Ollama, and other OpenAI API compatible AI providers. Wing 11 also adds package management with uv, improves Python code analysis, updates the UI localizations, improves diff/merge, adds easier custom key binding assignment, and much more.
Most of these improvements are available only in Wing Pro. Compare Products for details.
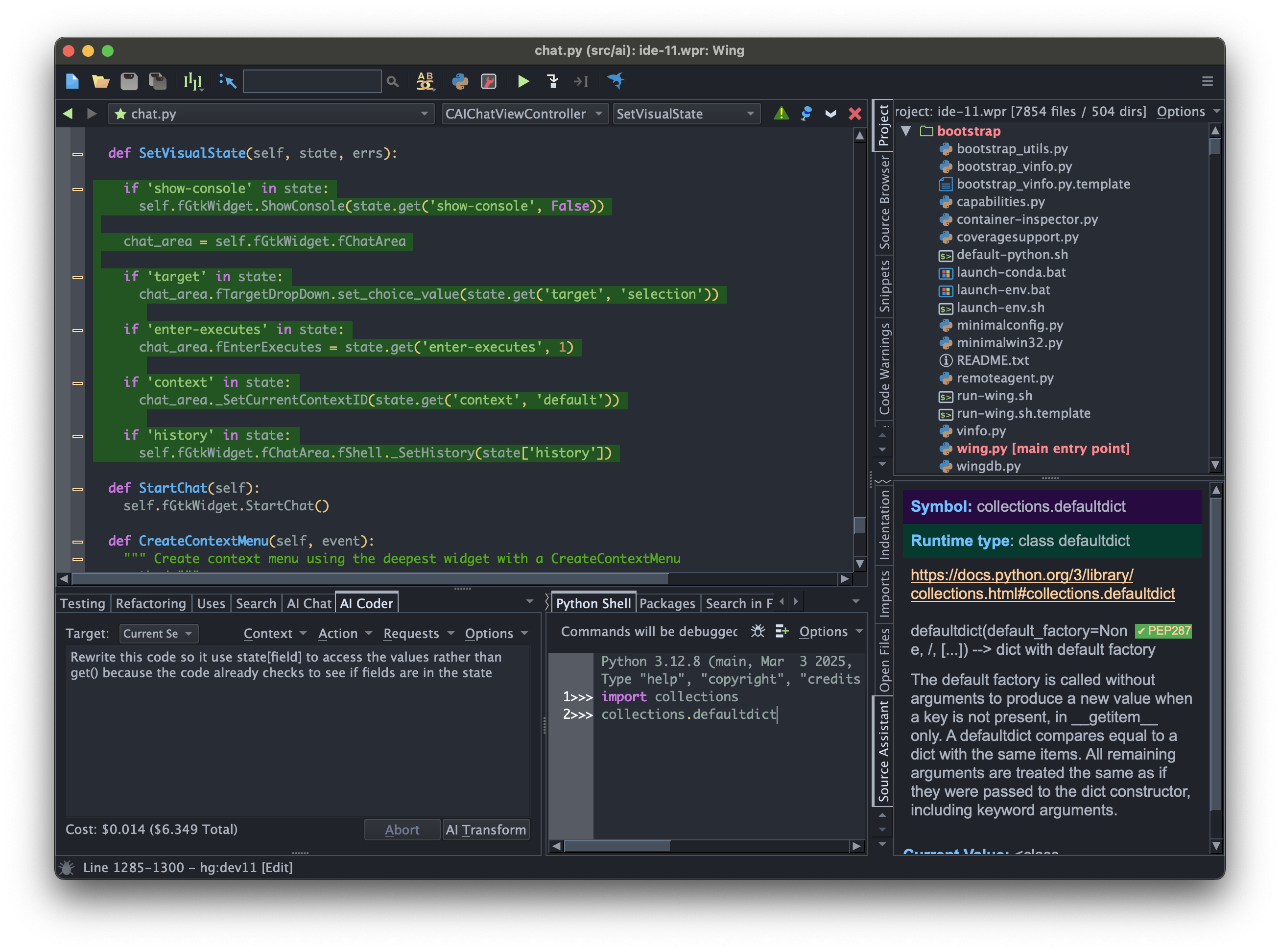
Downloads
Wing 10 and earlier versions are not affected by installation of Wing 11 and may be installed and used independently. However, project files for Wing 10 and earlier are converted when opened by Wing 11 and should be saved under a new name, since Wing 11 projects cannot be opened by older versions of Wing.
New in Wing 11
Improved AI Assisted Development
Wing 11 improves the user interface for AI assisted development by introducing two separate tools AI Coder and AI Chat. AI Coder can be used to write, redesign, or extend code in the current editor. AI Chat can be used to ask about code or iterate in creating a design or new code without directly modifying the code in an editor.
Wing 11's AI assisted development features now support not just OpenAI but also Claude, Grok, Gemini, Perplexity, Mistral, Deepseek, and any other OpenAI completions API compatible AI provider.
This release also improves setting up AI request context, so that both automatically and manually selected and described context items may be paired with an AI request. AI request contexts can now be stored, optionally so they are shared by all projects, and may be used independently with different AI features.
AI requests can now also be stored in the current project or shared with all projects, and Wing comes preconfigured with a set of commonly used requests. In addition to changing code in the current editor, stored requests may create a new untitled file or run instead in AI Chat. Wing 11 also introduces options for changing code within an editor, including replacing code, commenting out code, or starting a diff/merge session to either accept or reject changes.
Wing 11 also supports using AI to generate commit messages based on the changes being committed to a revision control system.
You can now also configure multiple AI providers for easier access to different models.
For details see AI Assisted Development under Wing Manual in Wing 11's Help menu.
Package Management with uv
Wing Pro 11 adds support for the uv package manager in the New Project dialog and the Packages tool.
For details see Project Manager > Creating Projects > Creating Python Environments and Package Manager > Package Management with uv under Wing Manual in Wing 11's Help menu.
Improved Python Code Analysis
Wing 11 improves code analysis of literals such as dicts and sets, parametrized type aliases, typing.Self, type variables on the def or class line that declares them, generic classes with [...], and __all__ in *.pyi files.
Updated Localizations
Wing 11 updates the German, French, and Russian localizations, and introduces a new experimental AI-generated Spanish localization. The Spanish localization and the new AI-generated strings in the French and Russian localizations may be accessed with the new User Interface > Include AI Translated Strings preference.
Improved diff/merge
Wing Pro 11 adds floating buttons directly between the editors to make navigating differences and merging easier, allows undoing previously merged changes, and does a better job managing scratch buffers, scroll locking, and sizing of merged ranges.
For details see Difference and Merge under Wing Manual in Wing 11's Help menu.
Other Minor Features and Improvements
Wing 11 also improves the custom key binding assignment user interface, adds a Files > Auto-Save Files When Wing Loses Focus preference, warns immediately when opening a project with an invalid Python Executable configuration, allows clearing recent menus, expands the set of available special environment variables for project configuration, and makes a number of other bug fixes and usability improvements.
Changes and Incompatibilities
Since Wing 11 replaced the AI tool with AI Coder and AI Chat, and AI configuration is completely different than in Wing 10, you will need to reconfigure your AI integration manually in Wing 11. This is done with Manage AI Providers in the AI menu. After adding the first provider configuration, Wing will set that provider as the default. You can switch between providers with Switch to Provider in the AI menu.
If you have questions, please don't hesitate to contact us at support@wingware.com.
Stéphane Wirtel
Ce que je fabrique pendant mes « vacances »
Ce que je fabrique pendant mes « vacances » Pour une fois, j’écris ce billet en français. Après tout, c’est ma langue maternelle, et j’ai envie de partager ce que je fais ces derniers temps avec un peu plus de spontanéité. Depuis fin avril, je suis en congé forcé. Mon contrat avec la Banque Européenne d’Investissement s’est terminé, et après deux années et demie de collaboration intense, passionnante et ultra-formante sur les sujets financiers et d’investissement, je me suis retrouvé avec… du temps.
June 04, 2025
Real Python
How to Find an Absolute Value in Python
Learn how to work with absolute values in Python using the built-in abs()
function for numbers, arrays, and custom objects. This tutorial shows you how to implement the absolute value function from scratch, use abs()
with numbers, and customize its behavior for data types like NumPy arrays and pandas Series.
By the end of this tutorial, you’ll understand that:
- You can implement the absolute value function in Python using conditional statements or mathematical operations.
- Python’s built-in
abs()
function efficiently handles integers, floating-point numbers, complex numbers, and more. - NumPy and pandas extend the
abs()
function to work directly with arrays and Series. - You can customize the behavior of
abs()
for your own data types by implementing the.__abs__()
method. - The
abs()
function can process fractions and decimals from Python’s standard library.
Don’t worry if your mathematical knowledge of the absolute value function is a little rusty. You’ll begin by refreshing your memory before diving deeper into Python code. That said, feel free to skip the next section and jump right into the nitty-gritty details that follow.
Get Your Code: Click here to download the free sample code that you’ll use to find absolute values in Python.
Take the Quiz: Test your knowledge with our interactive “How to Find an Absolute Value in Python” quiz. You’ll receive a score upon completion to help you track your learning progress:
Interactive Quiz
How to Find an Absolute Value in PythonIn this quiz, you'll test your knowledge of calculating absolute values in Python, mastering both built-in functions and common use cases to improve your coding accuracy.
Defining the Absolute Value
The absolute value lets you determine the size or magnitude of an object, such as a number or a vector, regardless of its direction. Real numbers can have one of two directions when you ignore zero: they can be either positive or negative. On the other hand, complex numbers and vectors can have many more directions.
Note: When you take the absolute value of a number, you lose information about its sign or, more generally, its direction.
Consider a temperature measurement as an example. If the thermometer reads -12°C, then you can say it’s twelve degrees Celsius below freezing. Notice how you decomposed the temperature in the last sentence into a magnitude, twelve, and a sign. The phrase below freezing means the same as below zero degrees Celsius. The temperature’s size or absolute value is identical to the absolute value of the much warmer +12°C.
Using mathematical notation, you can define the absolute value of 𝑥 as a piecewise function, which behaves differently depending on the range of input values. A common symbol for absolute value consists of two vertical lines:
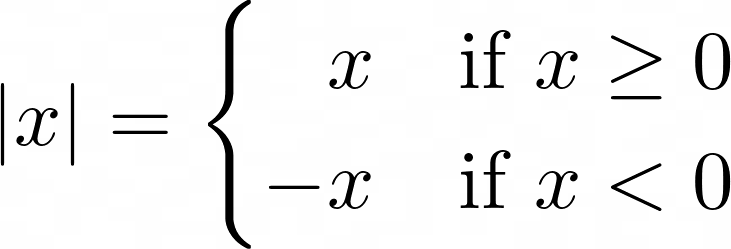
This function returns values greater than or equal to zero without alteration. On the other hand, values smaller than zero have their sign flipped from a minus to a plus. Algebraically, this is equivalent to taking the square root of a number squared:
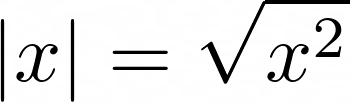
When you square a real number, you always get a positive result, even if the number that you started with was negative. For example, the square of -12 and the square of 12 have the same value, equal to 144. Later, when you compute the square root of 144, you’ll only get 12 without the minus sign.
Geometrically, you can think of an absolute value as the distance from the origin, which is zero on a number line in the case of the temperature reading from before:
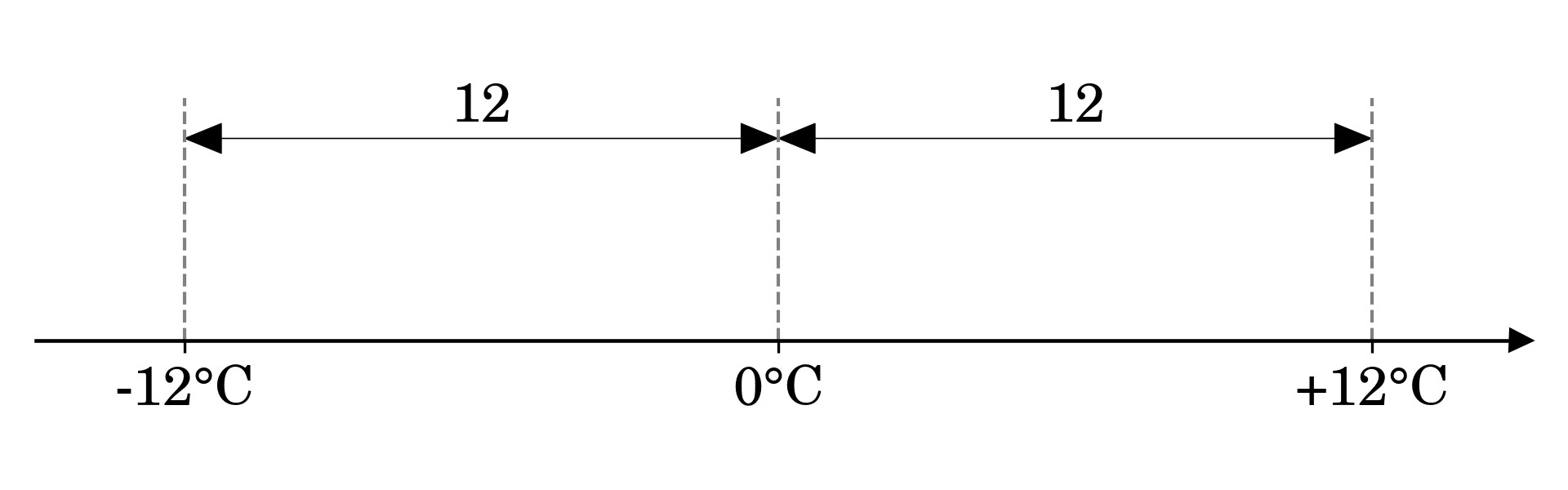
To calculate this distance, you can subtract the origin from the temperature reading (-12°C - 0°C = -12°C) or the other way around (0°C - (-12°C) = +12°C), and then drop the sign of the result. Subtracting zero doesn’t make much difference here, but the reference point may sometimes be shifted. That’s the case for vectors bound to a fixed point in space, which becomes their origin.
Vectors, just like numbers, convey information about the direction and the magnitude of a physical quantity, but in more than one dimension. For example, you can express the velocity of a falling snowflake as a three-dimensional vector:
This vector indicates the snowflake’s current position relative to the origin of the coordinate system. It also shows the snowflake’s direction and pace of motion through the space. The longer the vector, the greater the magnitude of the snowflake’s speed. As long as the coordinates of the vector’s initial and terminal points are expressed in meters, calculating its length will get you the snowflake’s speed measured in meters per unit of time.
Note: There are two ways to look at a vector. A bound vector is an ordered pair of fixed points in space, whereas a free vector only tells you about the displacement of the coordinates from point A to point B without revealing their absolute locations. Consider the following code snippet as an example:
>>> A = [1, 2, 3]
>>> B = [3, 2, 1]
>>> bound_vector = [A, B]
>>> bound_vector
[[1, 2, 3], [3, 2, 1]]
>>> free_vector = [b - a for a, b in zip(A, B)]
>>> free_vector
[2, 0, -2]
A bound vector wraps both points, providing quite a bit of information. In contrast, a free vector only represents the shift from A to B. You can calculate a free vector by subtracting the initial point, A, from the terminal one, B. One way to do so is by iterating over the consecutive pairs of coordinates with a list comprehension.
A free vector is essentially a bound vector translated to the origin of the coordinate system, so it begins at zero.
The length of a vector, also known as its magnitude, is the distance between its initial and terminal points, 𝐴 and 𝐵, which you can calculate using the Euclidean norm:
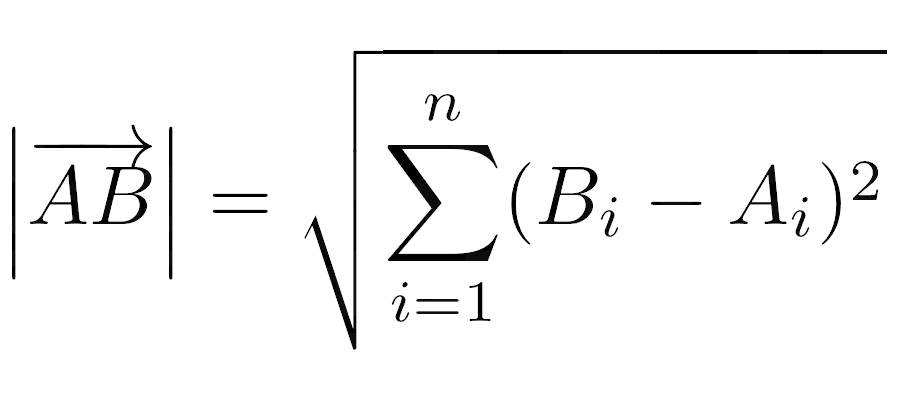
This formula calculates the length of the 𝑛-dimensional vector 𝐴𝐵, by summing the squares of the differences between the coordinates of points 𝐴 and 𝐵 in each dimension indexed by 𝑖. For a free vector, the initial point, 𝐴, becomes the origin of the coordinate system—or zero—which simplifies the formula, as you only need to square the coordinates of your vector.
Read the full article at https://realpython.com/python-absolute-value/ »
[ Improve Your Python With 🐍 Python Tricks 💌 – Get a short & sweet Python Trick delivered to your inbox every couple of days. >> Click here to learn more and see examples ]
Quiz: How to Find an Absolute Value in Python
In this quiz, you’ll test your understanding of How to Find an Absolute Value in Python.
By working through this quiz, you’ll revisit key concepts such as how to use Python’s built-in functions to compute absolute values, apply them in mathematical operations, and handle different data types effectively.
[ Improve Your Python With 🐍 Python Tricks 💌 – Get a short & sweet Python Trick delivered to your inbox every couple of days. >> Click here to learn more and see examples ]
Django Weblog
Django security releases issued: 5.2.2, 5.1.10, and 4.2.22
In accordance with our security release policy, the Django team is issuing releases for Django 5.2.2, Django 5.1.10, and Django 4.2.22. These releases address the security issues detailed below. We encourage all users of Django to upgrade as soon as possible.
CVE-2025-48432: Potential log injection via unescaped request path
Internal HTTP response logging used request.path directly, allowing control characters (e.g. newlines or ANSI escape sequences) to be written unescaped into logs. This could enable log injection or forgery, letting attackers manipulate log appearance or structure, especially in logs processed by external systems or viewed in terminals.
Although this does not directly impact Django's security model, it poses risks when logs are consumed or interpreted by other tools. To fix this, the internal django.utils.log.log_response() function now escapes all positional formatting arguments using a safe encoding.
Thanks to Seokchan Yoon (https://ch4n3.kr/) for the report.
This issue has severity "moderate" according to the Django security policy.
Affected supported versions
- Django main
- Django 5.2
- Django 5.1
- Django 4.2
Resolution
Patches to resolve the issue have been applied to Django's main, 5.2, 5.1, and 4.2 branches. The patches may be obtained from the following changesets.
CVE-2025-48432: Potential log injection via unescaped request path
- On the main branch
- On the 5.2 branch
- On the 5.1 branch
- On the 4.2 branch
The following releases have been issued
- Django 5.2.2 (download Django 5.2.2 | 5.2.2 checksums)
- Django 5.1.10 (download Django 5.1.10 | 5.1.10 checksums)
- Django 4.2.22 (download Django 4.2.22 | 4.2.22 checksums)
The PGP key ID used for this release is Natalia Bidart: 2EE82A8D9470983E
General notes regarding security reporting
As always, we ask that potential security issues be reported via private email to security@djangoproject.com, and not via Django's Trac instance, nor via the Django Forum. Please see our security policies for further information.
Armin Ronacher
AI Changes Everything
At the moment I'm working on a new project. Even over the last two months, the way I do this has changed profoundly. Where I used to spend most of my time in Cursor, I now mostly use Claude Code, almost entirely hands-off.
Do I program any faster? Not really. But it feels like I've gained 30% more time in my day because the machine is doing the work. I alternate between giving it instructions, reading a book, and reviewing the changes. If you would have told me even just six months ago that I'd prefer being an engineering lead to a virtual programmer intern over hitting the keys myself, I would not have believed it. I can go can make a coffee, and progress still happens. I can be at the playground with my youngest while work continues in the background. Even as I'm writing this blog post, Claude is doing some refactorings.
While all this is happening, I've found myself reflecting a lot on what AI means to the world and I am becoming increasingly optimistic about our future. It's obvious now that we're undergoing a tremendous shift. AI is out of the bottle, and there's no putting it back. Even if we halted all progress today, froze the weights, halted the training, the systems already out there would still reshape how we live, work, learn, and communicate to one another.
What however took longer to accept is just how profound that change really is. As an engineer coming from a world of deterministic things, who deeply values the craft of engineering, to accept the messiness of what agents are doing took a while to digest. It took me a while to even warm up to tool usage by AI in the first place — just two years ago I was convinced AI might kill my wife. In those two years however we've come incredibly far. We have reached the point where even if we stopped here (and there is no indication we will) AI is already a new substrate for a lot of new innovation, ideas and creations and I'm here for it. It has moved beyond being a curious tool.
Never before have I seen a technology surface in every day life so quickly, so widely. Smartphones adoption felt slow in comparison. Today I can't finish a commute or coffee without spotting someone chatting with ChatGPT. I've had conversations with baristas, hairdressers, parents at the playground — people who aren't what I would consider “tech-savvy” — telling me how AI changed the way they write letters, search for recipes, help their kids with homework, or translate documents. The ripple effect is already massive. And still, the majority of the world hasn't touched these tools yet. Entire communities, professions, and economies are yet to begin exploring their transformation.
That's what makes this moment feel so strange — half revolutionary, half prelude. And yet, oddly, there are so many technologists who are holdouts. How could techies reject this change? Thomas Ptacek's piece “My AI Skeptic Friends Are All Nuts” really resonated with me. It takes a humorous stab at the push against AI that is taking place from my very circles. Why is it that so many people I've respected in tech for years — engineers, open source contributors — are the ones most resistant to what's happening? We've built something beyond what we imagined, and instead of being curious, many are dismissive and denying its capabilities. What is that?
Of course the implications are vast and real and the rapid development forces big questions. What does this mean for the education of our children? If AI can teach, explain, and personalize lessons better than a classroom of thirty ever could, what becomes of schools as we know them? And if kids grow up expecting to interact with intelligence — not just absorb content — how do we teach them to reason, create, and collaborate in ways that leverage this power without becoming dependent on it?
On the global stage, there are also ramifications that seem more fundamental than in previous cycles. It does not feel like the rise of search engines or social media, where the rest of the world was satisfied with being a consumer of US infrastructure. This feels more like the invention of the steam engine. Once it existed, there was no future without it. No country could afford to stay on the sidelines. But steam machines also became quickly commoditized and there was plenty of competition of manufacturers. It was just too obvious of a technological leap. With AI, every nation, every corporation will want its own models, its own control, its own stake in the future.
And so, as I alternate between delegating tasks to Claude and reading something thoughtful in between, I can't help but feel excited about being there when we're at the beginning of something irreversible and expansive.
I understand why it's tempting to be cynical or fearful. For sure the job of programmers and artists will change, but they won't vanish. I feel like all my skills that I learned as a programmer are more relevant than ever, just with a new kind of tool. Likewise the abundance of AI generated art also makes me so much more determined that I want to hire an excellent designer as soon as I can. People will always value well crafted products. AI might raise the bar for everybody all at once, but it's the act of careful deliberation and very intentional creation that sets you apart from everybody else.
Sure, I may have good personal reasons to be optimistic. But the more time I spend with these tools, the more I believe that optimism is the more reasonable stance for everyone. AI can dramatically increase human agency when used well. It can help us communicate across cultures. It can democratize access to knowledge. It can accelerate innovation in medicine, science, and engineering.
Right now it's messy and raw, but the path is clear: we are no longer just using machines, we are now working with them. And while it's early, I think we'll look back at this decade the way past generations looked at electricity or the printing press — not as a curiosity, but as the moment when everything changed.
I encourage you not meet that moment with cynicism or fear: meet it with curiosity, responsibility and the conviction that this future will be bright and worth embracing.
June 03, 2025
PyCoder’s Weekly
Issue #684: NumPy Type Hints, LEGB, Pyrefly vs ty, and More (June 3, 2025)
#684 – JUNE 3, 2025
View in Browser »
NumPy Array Type Hints
Thanks to improvements in NumPy typing support, NumPy generic arrays can now type both shape
and dtype
, offering opportunities for improved static analysis and run-time validation.
CHRISTOPHER ARIZA • Shared by Christopher Ariza
The LEGB Rule & Understanding Python Scope
In this step-by-step video course, you’ll learn what scopes are, how they work, and how to use them effectively to avoid name collisions in your code.
REAL PYTHON course
Prevent Postgres Slowdowns on Python Apps with this Check List
Avoid performance regressions in your Python app by staying on top of Postgres maintenance. This monthly check list outlines what to monitor, how to catch slow queries early, and ways to ensure indexes, autovacuum, and I/O are performing as expected →
PGANALYZE sponsor
Pyrefly
vs. ty
: Comparing Python’s New Type Checkers
A deep dive on Python’s two newest, Rust-based type checkers. This post includes both performance and capability comparisons.
EDWARD LI
Discussions
Python Jobs
Sr. Software Developer (Python, Healthcare) (USA)
Senior Software Engineer – Quant Investment Platform (LA or Dallas) (Los Angeles, CA, USA)
Causeway Capital Management LLC
Articles & Tutorials
Journey Into Real Python
Amanda has recently written her first article for Real Python and this post talks about her experience doing so. If you want to check out the article, it is on Nested Loops.
AMANDA ENE ADOYI
Ways to Optimize Your Code in Python
Learn four ways to optimize your Python project and improve performance, including choosing the right data structures, variable scopes, list comprehensions and generators, and how to leverage built-in libraries.
FEDERICO TROTTA • Shared by AppSignal
DumPy: NumPy Except It’s OK if You’re Dum
In a follow-up to the article I Don’t Like NumPy, this post proposes a way of writing code that looks like loops but use vector operations instead.
DYNOMIGHT.NET
How to Split Up a Django Monolith Without Microservices
Facing too much complexity in your Django application as a developer? This articles introduces django-queuebie, a synchronous message queue working with commands and events.
RONNY VEDRILLA • Shared by Ronny Vedrilla
Control Flow Structures in Python
Take control of your code with Python control flow structures. You’ll learn with real examples using loops, conditionals, try-except blocks, and pattern matching.
REAL PYTHON
Python pandas Ditches NumPy for Speedier PyArrow
Pandas 3.0 will significantly boost performance by replacing NumPy with PyArrow as its default engine, enabling faster loading and reading of columnar data.
JOAB JACKSON
Writing Portable DataFrame Code for Lazy Execution
Learn one simple concept, and you can rid your code of row-ordering assumptions - it will then work seamlessly across eager and lazy DataFrames
MARCO GORELLI • Shared by Marco Gorelli
Marimo: A Reactive, Reproducible Notebook
Discover how marimo notebook simplifies coding with reactive updates, UI elements, and sandboxing for safe, sharable notebooks.
REAL PYTHON
Another Great PyCon
A post about the highlights of PyCon US 2025 from one of the volunteers.
GEORGI KER
Projects & Code
whatsonpypi: Check PyPI From the Command Line
GITHUB.COM/VISESHRP • Shared by visesh prasad
Events
Python Norte 2025
June 4 to June 7, 2025
PYTHONNORTE.ORG
Canberra Python Meetup
June 5, 2025
MEETUP.COM
Sydney Python User Group (SyPy)
June 5, 2025
SYPY.ORG
Django User Group Berlin
June 5, 2025
MEETUP.COM
AfroPython Conf 2025
June 7 to June 8, 2025
AFROPYTHONCONF.ORG
Django Girls Beira #1
June 7 to June 8, 2025
DJANGOGIRLS.ORG
Happy Pythoning!
This was PyCoder’s Weekly Issue #684.
View in Browser »
[ Subscribe to 🐍 PyCoder’s Weekly 💌 – Get the best Python news, articles, and tutorials delivered to your inbox once a week >> Click here to learn more ]
Python Insider
Python 3.13.4, 3.12.11, 3.11.13, 3.10.18 and 3.9.23 are now available
Python Release Party
It was only meant to be release day for 3.13.4 today, but poor number 13 looked so lonely… And hey, we had a couple of tarfile CVEs that we had to fix. So most of the Release Managers and all the Developers-in-Residence (including Security Developer-in-Residence Seth Michael Larson) came together to make it a full release party.
Security content in these releases
- gh-135034: Fixes multiple issues that allowed
tarfile
extraction filters (filter="data"
andfilter="tar"
) to be bypassed using crafted symlinks and hard links.Addresses CVE 2024-12718, CVE 2025-4138, CVE 2025-4330, and CVE 2025-4517. - gh-133767: Fix use-after-free in the “unicode-escape” decoder with a non-“strict” error handler.
- gh-128840: Short-circuit the processing of long IPv6 addresses early in
ipaddress
to prevent excessive memory consumption and a minor denial-of-service.
In addition to the security fixed mentioned above, a few additional changes to the ipaddress
were backported to make the security fixes feasible. (See the full changelogs for each release for more details.)
Python 3.13.4
In addition to the security fixes, the fourth maintenance release of Python 3.13 contains more than 300 bugfixes, build improvements and documentation changes.
https://www.python.org/downloads/release/python-3134/
Python 3.12.11
https://www.python.org/downloads/release/python-31211/
Python 3.11.13
https://www.python.org/downloads/release/python-31113/
Python 3.10.18
https://www.python.org/downloads/release/python-31018/
Python 3.9.23
Additional security content in this release (already fixed in older releases for the other versions):
- gh-80222: Fix bug in the folding of quoted strings when flattening an email message using a modern email policy. Previously when a quoted string was folded so that it spanned more than one line, the surrounding quotes and internal escapes would be omitted. This could theoretically be used to spoof header lines using a carefully constructed quoted string if the resulting rendered email was transmitted or re-parsed.
Stay safe and upgrade!
As always, upgrading is highly recommended to all users of affected versions.
Enjoy the new releases
Thanks to all of the many volunteers who help make Python Development and these releases possible! Please consider supporting our efforts by volunteering yourself or through organization contributions to the Python Software Foundation.
Regards from your very tired tireless release team,
Thomas Wouters
Pablo Galindo Salgado
Łukasz Langa
Ned Deily
Steve Dower
Sumana Harihareswara - Cogito, Ergo Sumana
Congratulating Leonard for his Community Service Award
Congratulating Leonard for his Community Service Award
Mirek Długosz
Interesting bugs: Artifact building failure caused by GitHub API rate limits
This is a story of a memorable bug I encountered at work. As with every story of that kind, there are a few ways to look at it: it might save you some time if you encounter a similar issue; it surfaces the hidden work - work that is not visible when you only look at the output and artifacts; it demonstrates the benefits of broad knowledge. I’m sharing it as a contribution to testing case library.
I was introducing a Jenkins pipeline to run for every pull request submitted to a project repository. One of the pipeline steps was to build a deployment artifact from the version of code with proposed changes. The artifact was a container image.
The application was following a standard architecture for web apps, with a separate backend and frontend communicating through an HTTP API. Each component had a separate repository hosted on GitHub and technically had an independent release policy that could result in version numbers that do not match. At the time, whole software was shipped as a single container image comprising both frontend and backend.
The container image was nominally a backend build artifact. One step in the container building procedure was to download the frontend files. The container build script recognized a special version “latest”, which caused script to ask GitHub API for latest releases and extract version of the newest one.
Not long after the pipeline was introduced, the team noticed that jobs sometimes fail at the container building step. Logs indicated GitHub responded with HTTP status 429 Too Many Requests. This happens when GitHub thinks that the client is exceeding the API rate limits.
However, the team was small and at the most active days created no more than a few pull requests. Even at the very unlucky timing, the combined pipeline runs from all pull requests should create no more than a dozen or two API requests in an hour. GitHub claimed to allow up to 60 requests per hour and it seemed extremely unlikely that we would routinely exceed that.
It should be noted that nobody in the team reported any problems with building containers locally. Developers were doing that for months already, often multiple times a day.
No matter how unlikely it seemed that our pipeline generated more than 60 requests per hour, that’s some clue - and one that is cheap to verify. I created a spreadsheet with one column to store the date of pipeline run, and another column to indicate if an error occurred or not. Assuming that rate limiting is the root cause, we would expect failures when there are several runs in the same one-hour window. The earlier ones would succeed, and later ones would fail. Runs that are far removed in time from other runs should never fail. Of course, this pattern was not found in the data.

The next step was to learn more about the GitHub API rate limits. When reading a documentation page on that topic, I learned there are multiple pools with different limits. 60 requests per hour is for unauthenticated users, grouped by IP address. Each authenticated user gets 5000 requests per hour.
At this point I need to share a bit of information about the wider infrastructure. This is subliminal context - something that you learn at some point when working in a company. It is not strictly related to your role and position, so it’s not something that you would think about constantly; but it’s a kind of background information you can draw from when thinking about issues that you face.
Jenkins controller was running inside a container deployed on OpenShift. Most of the work - including building deployment artifacts - was done on short-lived virtual machines provisioned on OpenStack. OpenShift and OpenStack operated from internal company network. The network was set up that outgoing connections to external world were allowed, but they all were routed through a limited number of proxy servers.
That allowed me to form a hypothesis - our pipeline is rate limited because from GitHub point of view, a sizable chunk of the company appears as a single IP address and is counted against a shared limit. It’s unlikely for our Jenkins agents to generate over 60 requests during normal operation, but all the development teams sharing OpenStack resources? I can see that happening.
This was not a research project, so I wasn’t exactly interested in refuting or corroborating that hypothesis. It’s enough that when stated like that, it provides clear steps towards solving the issue. If we authenticate our GitHub API requests, that should convince GitHub to count our requests against a different pool than the one shared with other OpenStack users. It will also increase our limit almost hundredfold, from 60 to 5000.
Requests may be authenticated by including a specific header with a token value. Token management, including ensuring they are not expired, is a separate topic I will not delve into here. In my case, the hardest part was working around the amount of layers that token value needs to be passed through. Jenkins created a virtual machine on OpenStack and provided GitHub API token value in environment variable. Pipeline at one point called make build-container
, which is basically a shortcut for more extensive podman build
command. Containerfile
copied Makefile
from backend project source directory to container build environment, and called make fetch-ui
there. That in turn used curl
to obtain data from the GitHub API. And this is where token needed to be used.

Child processes in Linux by default inherit the environment from their parent, so the basic solution is to ensure a specific environment variable is set by Jenkins and all the layers below indeed recognize it. Two things complicate the picture here.
First, the container build environment drops most of the surrounding environment variables. This is in part to ensure consistent and reproducible build environment. Variables that you want to use when creating a container need to be explicitly opted in.
The second thing is worse. Environment variables present in the build stage end up embedded in the final container image. Setting an environment variable fundamentally shares a value with all the people who have access to the container image. When it comes to things like access tokens, this becomes an unacceptable security liability.
Luckily, the problem was identified in the last few years and the container build tools now support secret mounting. They are special files that are available to containers at the build stage, but are exempted from final container image. It means that at one point after creating a Jenkins agent virtual machine, but before issuing an API request, the environment variable content needs to be written to a file, and that file must be passed as a secret to container build environment.
The final touch was ensuring that everything still works when an environment variable with a GitHub token is not set. Developers never experienced the problem locally and they shouldn’t be forced to maintain and set up a GitHub token in their own environment.
The final version of the solution I implemented at the time can be viewed in GitHub PR.
After merging the PR, we no longer observed pipeline failures at the container image building step caused by GitHub API rate limits. It means that the proposed solution turned out to be correct.
Real Python
Execute Your Python Scripts With a Shebang
In shell scripts, the shebang line (#!
) specifies the path to the interpreter that should execute the file. You can place it at the top of your Python file to tell the shell how to run your script, allowing you to execute the script directly without typing python
before the script name. The shebang is essential for Unix-like systems but ignored on Windows unless using specific compatibility layers.
By the end of this video course, you’ll understand that:
- A shebang specifies the path to the Python interpreter in scripts, allowing direct execution.
- You should include a shebang when a script needs direct execution, but not in import-only modules.
- Best practices for shebangs include using
/usr/bin/env
for portability and ensuring the script is executable. - Shebangs have limitations, such as being ignored on Windows without compatibility layers like WSL.
[ Improve Your Python With 🐍 Python Tricks 💌 – Get a short & sweet Python Trick delivered to your inbox every couple of days. >> Click here to learn more and see examples ]
Nicola Iarocci
Eve 2.2.1
Eve v2.2.1 was just released on PyPI. It is a minor upgrade, but it includes a remarkable performance increase contributed by Alexander Urieles. Also, thanks to Svante Bengtson and Pablo Parada for their help with this release.
Seth Michael Larson
Pikmin 2 International Treasure Hoard
Pikmin 2 is a treasure collect-a-thon for the GameCube. One of the most distinguishing features of the treasures is that they sometimes use real-life brands like Duracell and Dr. Pepper.
Pikmin 2 has three distinct regional treasure hoards, each with unique treasures: US, JP, and PAL. I've only personally played the US version of the game. I'd like to try collecting all 242 unique treasures across all three regions.
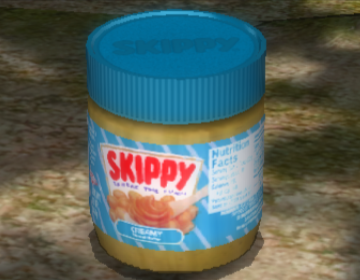
'Nutrient Silo', aka Skippy Peanut Butter in Pikmin 2
You can see my current progress below highlighted in green with timestamps for when I collected the treasure. So far I have 98/242 treasures collected (40%). This table is automatically generated as I collect more treasure (when playing on real GameCube hardware).
If you're on mobile I recommend scrolling left and right on the table to see all the columns.
Treasure | Regions | Location | Collected | |
---|---|---|---|---|
![]() | Spiny Alien Treat | Valley of Repose | Jun 04, 2025 22:49:31 | |
![]() | Fossilized Ursidae | Valley of Repose | ||
![]() | Utter Scrap | Valley of Repose | May 31, 2025 23:50:57 | |
![]() | Temporal Mechanism | Valley of Repose | Jun 04, 2025 22:49:31 | |
![]() | Unspeakable Wonder | Valley of Repose | Jun 04, 2025 22:57:48 | |
![]() | Pink Menace | Valley of Repose | Jun 04, 2025 22:49:31 | |
![]() | Courage Reactor | PAL, US | Valley of Repose | May 31, 2025 23:25:47 |
![]() | Citrus Lump | Emergence Cave | May 31, 2025 23:53:04 | |
![]() | Quenching Emblem | Emergence Cave | May 31, 2025 23:53:04 | |
![]() | Spherical Atlas | Emergence Cave | May 31, 2025 23:58:06 | |
![]() | Toxic Toadstool | White Flower Garden | Jun 01, 2025 09:27:40 | |
![]() | Superstick Textile | White Flower Garden | Jun 01, 2025 09:24:33 | |
![]() | Petrified Heart | White Flower Garden | Jun 01, 2025 09:21:29 | |
![]() | Survival Ointment | US | White Flower Garden | Jun 01, 2025 09:27:40 |
![]() | Alien Billboard | White Flower Garden | Jun 01, 2025 09:20:55 | |
![]() | Drought Ender | White Flower Garden | Jun 01, 2025 09:21:29 | |
![]() | Five-man Napsack | White Flower Garden | Jun 01, 2025 09:31:31 | |
![]() | Sunseed Berry | Awakening Wood | Jun 01, 2025 09:00:24 | |
![]() | Pilgrim Bulb | Awakening Wood | Jun 01, 2025 13:14:36 | |
![]() | Decorative Goo | Awakening Wood | Jun 01, 2025 15:50:59 | |
![]() | Air Brake | Awakening Wood | Jun 01, 2025 16:05:32 | |
![]() | Chance Totem | Awakening Wood | Jun 01, 2025 09:40:21 | |
![]() | Healing Cask | US | Awakening Wood | Jun 01, 2025 15:50:59 |
![]() | Geographic Projection | Awakening Wood | Jun 01, 2025 13:14:36 | |
![]() | Luck Wafer | Hole of Beasts | Jun 01, 2025 09:11:14 | |
![]() | Strife Monolith | Hole of Beasts | Jun 01, 2025 09:07:53 | |
![]() | Dream Architect | Hole of Beasts | Jun 01, 2025 09:11:14 | |
![]() | Stone of Glory | Hole of Beasts | Jun 01, 2025 09:03:29 | |
![]() | Cosmic Archive | Hole of Beasts | Jun 01, 2025 09:07:53 | |
![]() | Prototype Detector | Hole of Beasts | Jun 01, 2025 09:16:06 | |
![]() | Onion Replica | Perplexing Pool | ||
![]() | Fortified Delicacy | Perplexing Pool | ||
![]() | Impediment Scourge | PAL, US | Perplexing Pool | Jun 01, 2025 13:30:54 |
![]() | Massage Girdle | Perplexing Pool | ||
![]() | Aquatic Mine | Perplexing Pool | ||
![]() | Optical Illustration | US | Perplexing Pool | Jun 02, 2025 22:34:24 |
![]() | Gherkin Gate | PAL, US | Perplexing Pool | |
![]() | Love Nugget | Citadel of Spiders | Jun 01, 2025 13:33:15 | |
![]() | King of Sweets | Citadel of Spiders | Jun 01, 2025 15:24:52 | |
![]() | Memorial Shell | Citadel of Spiders | Jun 01, 2025 15:19:49 | |
![]() | Flame of Tomorrow | Citadel of Spiders | Jun 01, 2025 15:24:52 | |
![]() | Time Capsule | Citadel of Spiders | Jun 01, 2025 15:24:52 | |
![]() | Lip Service | PAL, US | Citadel of Spiders | Jun 01, 2025 13:39:07 |
![]() | Regal Diamond | Citadel of Spiders | Jun 01, 2025 15:32:40 | |
![]() | Paradoxical Enigma | Citadel of Spiders | Jun 01, 2025 13:39:07 | |
![]() | Patience Tester | PAL, US | Citadel of Spiders | Jun 01, 2025 15:19:49 |
![]() | Creative Inspiration | US | Citadel of Spiders | Jun 01, 2025 13:39:07 |
![]() | The Key | Citadel of Spiders | Jun 01, 2025 15:32:40 | |
![]() | Meat of Champions | Glutton's Kitchen | Jun 03, 2025 11:41:02 | |
![]() | Hideous Victual | Glutton's Kitchen | Jun 03, 2025 11:41:02 | |
![]() | Imperative Cookie | Glutton's Kitchen | Jun 03, 2025 11:21:46 | |
![]() | White Goodness | Glutton's Kitchen | Jun 03, 2025 11:29:01 | |
![]() | Sweet Dreamer | Glutton's Kitchen | Jun 03, 2025 11:41:02 | |
![]() | Invigorator | Glutton's Kitchen | Jun 03, 2025 11:29:01 | |
![]() | Master's Instrument | Glutton's Kitchen | Jun 03, 2025 11:17:40 | |
![]() | Harmonic Synthesizer | Glutton's Kitchen | Jun 03, 2025 11:25:38 | |
![]() | Director of Destiny | Glutton's Kitchen | Jun 03, 2025 11:25:38 | |
![]() | Sulking Antenna | Glutton's Kitchen | Jun 03, 2025 11:33:08 | |
![]() | Boom Cone | US | Glutton's Kitchen | Jun 03, 2025 11:33:08 |
![]() | Massive Lid | US | Glutton's Kitchen | Jun 03, 2025 11:21:46 |
![]() | Happiness Emblem | US | Glutton's Kitchen | Jun 03, 2025 11:29:01 |
![]() | Dream Material | Glutton's Kitchen | Jun 03, 2025 11:41:02 | |
![]() | Anxious Sprout | Bulblax Kingdom | Jun 02, 2025 22:09:49 | |
![]() | Colossal Fossil | Bulblax Kingdom | Jun 02, 2025 22:15:54 | |
![]() | Olimarnite Shell | Bulblax Kingdom | Jun 02, 2025 22:02:09 | |
![]() | Crystal King | Bulblax Kingdom | Jun 02, 2025 22:07:30 | |
![]() | Gyroid Bust | Bulblax Kingdom | Jun 02, 2025 22:20:43 | |
![]() | Unknown Merit | Bulblax Kingdom | Jun 02, 2025 22:07:30 | |
![]() | Eternal Emerald Eye | Bulblax Kingdom | Jun 02, 2025 22:15:54 | |
![]() | Tear Stone | Bulblax Kingdom | Jun 02, 2025 22:00:05 | |
![]() | Crystal Clover | Bulblax Kingdom | Jun 02, 2025 21:57:59 | |
![]() | Forged Courage | Bulblax Kingdom | Jun 02, 2025 22:20:43 | |
![]() | Cupid's Grenade | Snagret Hole | Jun 01, 2025 16:24:58 | |
![]() | Combustion Berry | Snagret Hole | Jun 01, 2025 16:13:52 | |
![]() | Science Project | Snagret Hole | Jun 01, 2025 16:55:19 | |
![]() | Meat Satchel | Snagret Hole | Jun 01, 2025 16:18:54 | |
![]() | Taste Sensation | Snagret Hole | Jun 01, 2025 16:13:52 | |
![]() | Triple Sugar Threat | Snagret Hole | Jun 01, 2025 16:55:19 | |
![]() | Leviathan Feather | Snagret Hole | Jun 01, 2025 16:08:04 | |
![]() | Heavy-Duty Magnetizer | Snagret Hole | Jun 01, 2025 16:24:58 | |
![]() | Emperor Whistle | Snagret Hole | Jun 01, 2025 16:28:46 | |
![]() | Crystallized Telepathy | Snagret Hole | Jun 01, 2025 16:24:58 | |
![]() | Crystallized Telekinesis | Snagret Hole | Jun 01, 2025 16:08:04 | |
![]() | Crystallized Clairvoyance | Snagret Hole | Jun 01, 2025 16:28:46 | |
![]() | Stupendous Lens | US | Snagret Hole | Jun 01, 2025 16:55:19 |
![]() | Salivatrix | PAL, US | Snagret Hole | Jun 01, 2025 16:55:19 |
![]() | Justice Alloy | Snagret Hole | Jun 01, 2025 17:02:07 | |
![]() | Mystical Disc | Subterranean Complex | Jun 05, 2025 11:41:20 | |
![]() | Vacuum Processor | Subterranean Complex | Jun 05, 2025 11:48:24 | |
![]() | Indomitable CPU | Subterranean Complex | Jun 05, 2025 11:54:11 | |
![]() | Network Mainbrain | Subterranean Complex | Jun 04, 2025 23:09:01 | |
![]() | Space Wave Receiver | Subterranean Complex | Jun 05, 2025 11:48:24 | |
![]() | Nouveau Table | Subterranean Complex | Jun 04, 2025 23:02:53 | |
![]() | Omega Flywheel | Subterranean Complex | Jun 05, 2025 11:33:36 | |
![]() | Spirit Flogger | Subterranean Complex | Jun 04, 2025 23:09:01 | |
![]() | Superstrong Stabilizer | Subterranean Complex | Jun 05, 2025 11:33:36 | |
![]() | Repair Juggernaut | Subterranean Complex | Jun 05, 2025 11:48:24 | |
![]() | Adamantine Girdle | Subterranean Complex | Jun 05, 2025 11:41:20 | |
![]() | Exhausted Superstick | Subterranean Complex | Jun 04, 2025 23:02:53 | |
![]() | Furious Adhesive | Subterranean Complex | Jun 05, 2025 11:54:11 | |
![]() | Coiled Launcher | Subterranean Complex | Jun 05, 2025 11:33:36 | |
![]() | Thirst Activator | US | Subterranean Complex | Jun 05, 2025 11:54:11 |
![]() | Stellar Orb | Subterranean Complex | Jun 05, 2025 12:00:23 | |
![]() | Joy Receptacle | Frontier Cavern | ||
![]() | Fleeting Art Form | Frontier Cavern | ||
![]() | Danger Chime | Frontier Cavern | ||
![]() | Spouse Alert | Frontier Cavern | ||
![]() | Innocence Lost | Frontier Cavern | ||
![]() | Essential Furnishing | Frontier Cavern | ||
![]() | Icon of Progress | Frontier Cavern | ||
![]() | Essence of Rage | Frontier Cavern | ||
![]() | Gemstar Husband | Frontier Cavern | ||
![]() | Omniscient Sphere | Frontier Cavern | ||
![]() | Flame Tiller | Frontier Cavern | ||
![]() | Worthless Statue | Frontier Cavern | ||
![]() | Priceless Statue | Frontier Cavern | ||
![]() | Brute Knuckles | Frontier Cavern | ||
![]() | Repugnant Appendage | Frontier Cavern | ||
![]() | Arboreal Frippery | JP, US | Shower Room | |
![]() | Scrumptious Shell | Shower Room | ||
![]() | Vorpal Platter | Shower Room | ||
![]() | Merciless Extractor | PAL, US | Shower Room | |
![]() | Broken Food Master | Shower Room | ||
![]() | Sud Generator | Shower Room | ||
![]() | Mirrored Stage | Shower Room | ||
![]() | Behemoth Jaw | Shower Room | ||
![]() | Rubber Ugly | Shower Room | ||
![]() | Durable Energy Cell | PAL, US | Shower Room | |
![]() | Endless Repository | PAL, US | Shower Room | |
![]() | Abstract Masterpiece | US | Shower Room | |
![]() | Pondering Emblem | US | Shower Room | |
![]() | Amplified Amplifier | Shower Room | ||
![]() | Compelling Cookie | Submerged Castle | ||
![]() | Bug Bait | Submerged Castle | ||
![]() | Comfort Cookie | Submerged Castle | ||
![]() | Succulent Mattress | Submerged Castle | ||
![]() | Diet Doomer | Submerged Castle | ||
![]() | Pale Passion | Submerged Castle | ||
![]() | Chocolate Cushion | Submerged Castle | ||
![]() | Confection Hoop | Submerged Castle | ||
![]() | Pastry Wheel | Submerged Castle | ||
![]() | Proton AA | PAL, US | Submerged Castle | |
![]() | Drone Supplies | US | Submerged Castle | |
![]() | Activity Arouser | US | Submerged Castle | |
![]() | Professional Noisemaker | Submerged Castle | ||
![]() | Seed of Greed | Wistful Wild | ||
![]() | Anti-hiccup Fungus | Wistful Wild | ||
![]() | Conifer Spire | Wistful Wild | ||
![]() | Armored Nut | Wistful Wild | ||
![]() | Doomsday Apparatus | Wistful Wild | ||
![]() | Child of the Earth | Cavern of Chaos | ||
![]() | Infernal Vegetable | Cavern of Chaos | ||
![]() | Growshroom | Cavern of Chaos | ||
![]() | Impenetrable Cookie | Cavern of Chaos | ||
![]() | Enamel Buster | Cavern of Chaos | ||
![]() | Mysterious Remains | PAL, US | Cavern of Chaos | |
![]() | Milk Tub | Cavern of Chaos | ||
![]() | Princess Pearl | Cavern of Chaos | ||
![]() | Essence of Despair | Cavern of Chaos | ||
![]() | Frosty Bauble | Cavern of Chaos | ||
![]() | Gemstar Wife | Cavern of Chaos | ||
![]() | Mirth Sphere | Cavern of Chaos | ||
![]() | Maternal Sculpture | Cavern of Chaos | ||
![]() | Silencer | Cavern of Chaos | ||
![]() | Wiggle Noggin | Cavern of Chaos | ||
![]() | Fuel Reservoir | PAL, US | Cavern of Chaos | |
![]() | Fruit Guard | US | Cavern of Chaos | |
![]() | Corpulent Nut | Hole of Heroes | ||
![]() | Lustrous Element | Hole of Heroes | ||
![]() | Dimensional Slicer | US | Hole of Heroes | |
![]() | Essence of True Love | Hole of Heroes | ||
![]() | Joyless Jewel | Hole of Heroes | ||
![]() | Love Sphere | Hole of Heroes | ||
![]() | Remembered Old Buddy | Hole of Heroes | ||
![]() | Fond Gyro Block | Hole of Heroes | ||
![]() | Memorable Gyro Block | Hole of Heroes | ||
![]() | Lost Gyro Block | Hole of Heroes | ||
![]() | Favorite Gyro Block | Hole of Heroes | ||
![]() | Treasured Gyro Block | Hole of Heroes | ||
![]() | Nutrient Silo | PAL, US | Hole of Heroes | |
![]() | Disguised Delicacy | Dream Den | ||
![]() | Insect Condo | Dream Den | ||
![]() | Possessed Squash | Dream Den | ||
![]() | Future Orb | Dream Den | ||
![]() | Mirrored Element | Dream Den | ||
![]() | Manual Honer | Dream Den | ||
![]() | Implement of Toil | Dream Den | ||
![]() | Essence of Desire | Dream Den | ||
![]() | Universal Com | Dream Den | ||
![]() | Extreme Perspirator | Dream Den | ||
![]() | Boss Stone | Dream Den | ||
![]() | Talisman of Life | Dream Den | ||
![]() | Glee Spinner | Dream Den | ||
![]() | Stringent Container | PAL, US | Dream Den | |
![]() | Yellow Taste Tyrant | PAL, US | Dream Den | |
![]() | Hypnotic Platter | US | Dream Den | |
![]() | Shock Therapist | Dream Den | ||
![]() | Flare Cannon | Dream Den | ||
![]() | Comedy Bomb | Dream Den | ||
![]() | Monster Pump | Dream Den | ||
![]() | King of Bugs | Dream Den | ||
![]() | Love and Courage Reactor | JP | Valley of Repose | Jun 05, 2025 14:09:04 |
![]() | Paper Slider | JP | White Flower Garden | |
![]() | Seat of Enlightenment | JP | Awakening Wood | |
![]() | Lightning Bolt | JP | Perplexing Pool | |
![]() | Yell Battery | JP | Perplexing Pool | |
![]() | Idea Assistant | JP | Perplexing Pool | |
![]() | Family Raft | JP | Citadel of Spiders | |
![]() | Open Architecture | JP, PAL | Citadel of Spiders | |
![]() | Milky Team | JP | Citadel of Spiders | |
![]() | Magical Stage | JP, PAL | Glutton's Kitchen | |
![]() | Milk Lid | JP | Glutton's Kitchen | |
![]() | Good Old Memories | JP | Glutton's Kitchen | |
![]() | Ultimate Spinner | JP | Snagret Hole | |
![]() | Coiny The Refined | JP | Snagret Hole | |
![]() | Alternative Reactor | JP | Subterranean Complex | |
![]() | Broken Cooking God | JP | Shower Room | |
![]() | Revised Eternal Fuel Dynamo | JP | Shower Room | |
![]() | Permanent Container | JP, PAL | Shower Room | |
![]() | Estimated Object GF | JP | Shower Room | |
![]() | Milky Cradle | JP | Shower Room | |
![]() | Proton X | JP | Submerged Castle | |
![]() | Open Archive | JP, PAL | Submerged Castle | |
![]() | Anywhere Floater | JP | Submerged Castle | |
![]() | Omegatron | JP | Cavern of Chaos | |
![]() | Empty Space Container | JP, PAL | Cavern of Chaos | |
![]() | Understood Person Symbol | JP | Cavern of Chaos | |
![]() | Stringent Container | JP, PAL | Hole of Heroes | |
![]() | Flying Saucer | JP | Hole of Heroes | |
![]() | Endless Repository | JP, PAL | Dream Den | |
![]() | Universally Best Art | JP | Dream Den | |
![]() | Inviting...Thing | JP | Dream Den | |
![]() | Container of Sea Bounty | PAL | White Flower Garden | |
![]() | Hypnotic Platter | PAL | Awakening Wood | |
![]() | Abstract Masterpiece | PAL | Perplexing Pool | |
![]() | Activity Arouser | PAL | Citadel of Spiders | |
![]() | Drone Supplies | PAL | Glutton's Kitchen | |
![]() | Arboreal Frippery | PAL | Shower Room | |
![]() | Plentiful Tank | PAL | Shower Room | |
![]() | Happiness Emblem | PAL | Shower Room | |
![]() | Pondering Emblem | PAL | Submerged Castle | |
![]() | Container of Knowledge | PAL | Cavern of Chaos |
Generating the table
The table is laid out in an approximate order for collection taking into account the region(s) the treasure is available in and the location in-game. I already own the US region copy of Pikmin 2, I've purchased a JP region copy from eBay, and the PAL region is on my wishlist.
You can see that at a minimum to collect every treasure you need to complete the Holes of Heroes and the Dream Den (final two caves of Pikmin 2) in both US and JP, but neither cave has region-exclusive treasure in PAL. This means it's probably optimal to leave PAL for the last playthrough.
The above treasure hoard was generated by copying the save data on my
Memcard Pro GC regularly over time via FTP. The script to generate the table above
from a directory of memory card dumps (.raw
files)
is available on GitHub.
June 02, 2025
Quansight Labs Blog
There are lots of accessibility resources
Complications of having all these resources and methods to manage them.
Ari Lamstein
New Release: Covid Demographics Explorer v2
I recently published a new version of my Covid Demographics Explorer app. I encourage you to try it out!
This version adds data from the 2023 American Community Survey (ACS) 1-year estimates, and has several other improvements.
Updated Visualizations
People who saw my talk about this project know what led me to start it: during Covid the apartment building I live in essentially emptied out. So many people left the building that I felt self-conscious that I had stayed. I wanted to compare my experience during Covid to official statistics.
The first graph in the app still defaults to a time series of San Francisco’s population. Although now there is a dashed line to indicate that data from 2020 is missing. I also use color to highlight which years are pre- and post-Covid:
The official statistics generally match my recollection: SF had a large population drop during Covid. What surprises me is that the population hasn’t returned to pre-Covid levels (to me, San Francisco feels as busy as it did pre-Covid).
After seeing this graph people often make two comments:
- This graph makes SF’s post-Covid population drop seem dramatic. Perhaps if you expressed the change on a percentage basis it would seem smaller.
- Lots of counties experienced a population drop during Covid. Can you compare what happened in SF to what happened elsewhere?
I wanted to create a single visualization that would answer both of these questions. I tried several things but was not happy with the results. Then on LinkedIn Brent Brewington recommended I try a “Swarm Plot”. I had never heard of this visualization before, but I think it is the right tool for the job. (If you like technical discussions like this then please follow me on LinkedIn.)
In the swarm plot below each county is represented by a dot. Its position along the x-axis indicates how its population has changed, on a percentage basis, since Covid. The y-axis is meaningless: it’s just used to prevent the dots from overlapping. Because San Francisco appears so far to the left, you can see that it’s an outlier. In fact, of the 813 counties in this dataset, only two had a larger decrease.
Another Example
Taken together, this pair of graphs is powerful. They allow you to quickly see not just how a county changed, but also how that change compares to how every other county changed.
Consider the number of people who work from home. Between 2019 and 2023 San Francisco saw a 183% increase in the number of people who work from home. This is a big increase! But as the swarm plot shows, this change is similar to what happened in most other counties.
Technical Changes
The initial version of the Covid Demographics Explorer, which I released last summer, was the first project I completed in Python. One year later, I’ve grown a lot technically. So when I reviewed the code, I saw opportunities to improve it.
Continuous Integration (CI)
Last fall I took Matt Harrison’s Professional Python course. One week we did a deep dive into Python’s ecosystem around linting. We started by learning about tools like black
, flake8
and ruff
. Then we learned that it’s popular to run these tools on every Pull Request using GitHub Actions.
Longtime readers will know that shortly after taking Professional Python I began contributing to the censusdis
package. That repo uses these tools in the same way that Matt taught them. So I decided to set up a CI workflow for the project’s repo to do the same thing.
If you’re interested in setting up something similar, a good place to start is my repo’s lint.yml file. First copy it to the same path in your own repo. Then enable GitHub Actions on the repo.
uv vs. requirements.txt
The initial version of this project used pip
and requirements.txt
to list its dependencies. This is still probably the most popular way to manage project dependencies in Python.
But in Professional Python we learned about uv
, a newer and faster way to manage Python environments. uv has only grown in popularity since I took Professional Python – I continue to be amazed at how frequently I hear it talked about at meetups and on LinkedIn. So I decided to port this project to uv as well.
If you’d like help getting started with uv, check out the instructions I wrote in DEVELOPER.md. They explain how to install uv locally and recreate my virtual environment. The final step is running the app locally – if that works, then everything was set up correctly.
Directory Structure
This version increased the number of files in the project quite a bit. One way I addressed this is by putting all the files related to data generation in a separate subdirectory. This failed because the data generation script tried to import a function from a file one level up (in the home directory). I kept on getting errors like ImportError: attempted relative import with no known parent package
.
This seemed like such a common thing to do, I assumed that I must be missing something obvious. But after reading this StackOverflow question (which has 2.1 million views!) I began to realize that this is a very common problem Python programmers have. Some of the comments (such as this one) express a lot of frustration at how hard Python makes this.
In case it helps someone with a similar problem, here is what I wrote in my PR that fixed the issue:
Remove dependencies data directory had on its parent directory.It appears that the way Python works, life is easier if a script in a subdirectory (such as data/gen_county_data.py) does not import anything from a parent directory. Note that gen_county_data.py is a script (meaning it is meant to be run on the command line), and not a module (meaning it is not meant to be imported into another script).
In practice this meant moving the get_unique_census_labels function from /backend.py to /data/census_vars.py.
This required turning the data directory into a package (i.e. adding a __init__.py file to it) so that the function could be accessed by modules in the parent directory.
This allows me to continue to run the data generation script as “python gen_county_data.py” from the command line in the directory where it exists. It appears that the old design might have required me to run the script from a directory above and as a module (i.e. with “python -m”).
Migration to censusdis v1.4.0
Early in the development of this project I learned a hard lesson about ACS data: variables can change meaning over time. For example, Census currently uses variable B08006_017E to count the number of people who work from home. But in 2005 they used that variable to count the number of people who commute by motorcycle! (That year they used B08006_021E to count people who work from home).
In the initial version of the app I added a lot of code to my data generation script to catch errors where a variable unexpectedly changes meaning over time. A few months ago I contributed that code to the censusdis
package, so that others can easily use it.
In this version of the Covid Demographics Explorer I refactored the data generation script to use the error checking code that’s now in censusdis. The result was pretty significant: in addition to deleting an entire module, the data generation script went from 130 to 69 lines. Even better, I think the script is now much easier to read (link).
Closing Thoughts
First of all, I hope that this app helps you better understand how your county has changed since Covid. I personally found that looking at Census data for San Francisco, and comparing it to what happened in other counties, gave me a new perspective on how Covid changed my city.
Second of all, this project is open source and released under a very permissive license. I hope that the app inspires at least one person to modify it to answer their own questions about how America has changed over time. If you wind up using it as an starting off point for another project, please drop me a line!
Finally, if you enjoy the app, please consider giving the repo a star on github! I just started a job search, and heard that having “popular” repos on github can help with that.
While comments on my blog are closed, I welcome hearing from readers. You can contact me here.
Real Python
How Can You Structure Your Python Script?
You may have begun your Python journey interactively, exploring ideas within Jupyter Notebooks or through the Python REPL. While that’s great for quick experimentation and immediate feedback, you’ll likely find yourself saving code into .py
files. However, as your codebase grows, your Python script structure efficiency becomes increasingly important.
Transitioning from interactive environments to structured scripts helps promote readability, enabling better collaboration and more robust development practices. This tutorial transforms messy scripts into well-organized, shareable code. Along the way, you’ll learn standard Python practices and tools. These techniques bridge the gap between quick scripting and disciplined software development.
By the end of this tutorial, you’ll know how to:
- Organize your Python scripts logically with functions, constants, and appropriate import practices.
- Efficiently manage your script’s state using data structures such as enumerations and data classes.
- Enhance interactivity through command-line arguments and improve robustness with structured feedback using logging and libraries like Rich.
- Create self-contained, shareable scripts by handling dependencies inline using PEP 723.
Without further ado, it’s time to start working through a concrete script that interacts with a web server to obtain and manipulate a machine learning dataset.
Get Your Code: Click here to download the free sample code you’ll use to learn how you can structure your Python script.
Take the Quiz: Test your knowledge with our interactive “How Can You Structure Your Python Script?” quiz. You’ll receive a score upon completion to help you track your learning progress:
Interactive Quiz
How Can You Structure Your Python Script?In this quiz, you'll test your understanding of organizing and structuring Python scripts. You'll revisit key concepts about best practices for writing clear, maintainable, and executable Python code.
Setting the Stage for Scripting
Throughout this tutorial, you’ll apply the structuring concepts by building a Python script step-by-step. The goal of this script will be to work with the well-known Iris dataset, a classic dataset in machine learning containing measurements for three species of Iris flowers.
Your script, called iris_summary.py
, will evolve through several stages, demonstrating different structural improvements. These stages are:
-
Set Up the Initial Script: Begin with a functional script using standard language features. Apply a foundational structure using named constants for clarity and the entry-point guard to separate executable code from importable definitions.
-
Integrate External Libraries and Dependencies: Incorporate third-party libraries when needed to leverage specialized functionality or simplify complex tasks. Declare and manage script dependencies within the file using standards like PEP 723 for better reproducibility.
-
Handle Command-Line Arguments: Add command-line arguments using helper libraries to make the script interactive and configurable. Define a clear
main()
function to encapsulate the core script logic triggered by the command-line interface (CLI). -
Structure Internal Data: Improve how data is represented by selecting appropriate data structures. Move beyond basic types and use constructs like
enum
for fixed choices, ordataclass
andnamedtuple
for structured records. -
Enhance Feedback and Robustness: Refine how the script communicates its progress and results. Implement structured logging instead of relying solely on
print()
. Useassert
statements for internal consistency checks during development, and improve the terminal output presentation, potentially using libraries designed for richer interfaces, like Rich.
By following these steps, you’ll see how structure transforms a basic script into something more robust, readable, and shareable. Each new concept will be introduced and immediately applied to the evolving Iris script.
Before diving into the specifics of script structure, it’s important to understand some foundational elements that make your Python scripts executable and well-organized.
Using the Shebang Line
On Unix-like systems, such as Linux and macOS, you can make your Python script directly executable from the command line, like ./iris_summary.py
, instead of always typing python iris_summary.py
. This involves making the file executable with chmod +x iris_summary.py
, and adding a shebang line at the top of your file.
The shebang tells the system which interpreter to use. The recommended, portable shebang for Python is:
#!/usr/bin/env python3
# Your script logic goes here...
This small addition signals that your file is intended to be run as a standalone script.
Note: The dedicated tutorial Executing Python Scripts With a Shebang provides a comprehensive look at how a shebang works, why /usr/bin/env
is used, how to handle arguments, and how to account for platform differences.
Now that you know how to tell the operating system how to run your script, you can focus on organizing the code within the script, starting with imports.
Organizing the Import Statements
As your script starts interacting with more modules, the import
statements at the top of your file become important for clarity and code quality. Python’s official style guide, PEP 8, recommends specific conventions for ordering imports, which significantly improves readability. Following these conventions is standard practice, and there are modern tools like Ruff to enforce these conventions.
Following a standard order helps anyone reading your code quickly understand its dependencies. The recommended grouping is:
- Standard Library Imports: Modules included with Python, like
pathlib
. - Third-Party Imports: Libraries you’ve installed with
pip
, likerequests
. - Local Imports: Local modules, either application files or libraries, such as when importing another
.py
file you wrote.
A good scripting practice for sharing code is to avoid local or library-specific imports and to ensure that only cross-platform third-party packages are used.
Read the full article at https://realpython.com/python-script-structure/ »
[ Improve Your Python With 🐍 Python Tricks 💌 – Get a short & sweet Python Trick delivered to your inbox every couple of days. >> Click here to learn more and see examples ]
Talk Python to Me
#507: Agentic AI Workflows with LangGraph
If you want to leverage the power of LLMs in your Python apps, you would be wise to consider an agentic framework. Agentic empowers the LLMs to use tools and take further action based on what it has learned at that point. And frameworks provide all the necessary building blocks to weave these into your apps with features like long-term memory and durable resumability. I'm excited to have Sydney Runkle back on the podcast to dive into building Python apps with LangChain and LangGraph.<br/> <br/> <strong>Episode sponsors</strong><br/> <br/> <a href='https://talkpython.fm/ppm'>Posit</a><br> <a href='https://talkpython.fm/auth0'>Auth0</a><br> <a href='https://talkpython.fm/training'>Talk Python Courses</a><br/> <br/> <h2 class="links-heading">Links from the show</h2> <div><strong>Sydney Runkle</strong>: <a href="https://www.linkedin.com/in/sydney-runkle-105a35190/?featured_on=talkpython" target="_blank" >linkedin.com</a><br/> <strong>LangGraph</strong>: <a href="https://github.com/langchain-ai/langgraph?featured_on=talkpython" target="_blank" >github.com</a><br/> <strong>LangChain</strong>: <a href="https://www.langchain.com/?featured_on=talkpython" target="_blank" >langchain.com</a><br/> <strong>LangGraph Studio</strong>: <a href="https://github.com/langchain-ai/langgraph-studio?featured_on=talkpython" target="_blank" >github.com</a><br/> <strong>LangGraph (Web)</strong>: <a href="https://www.langchain.com/langgraph?featured_on=talkpython" target="_blank" >langchain.com</a><br/> <strong>LangGraph Tutorials Introduction</strong>: <a href="https://langchain-ai.github.io/langgraph/tutorials/introduction/?featured_on=talkpython" target="_blank" >langchain-ai.github.io</a><br/> <strong>How to Think About Agent Frameworks</strong>: <a href="https://blog.langchain.dev/how-to-think-about-agent-frameworks/?featured_on=talkpython" target="_blank" >blog.langchain.dev</a><br/> <strong>Human in the Loop Concept</strong>: <a href="https://langchain-ai.github.io/langgraph/concepts/human_in_the_loop/?featured_on=talkpython" target="_blank" >langchain-ai.github.io</a><br/> <strong>GPT-4 Prompting Guide</strong>: <a href="https://cookbook.openai.com/examples/gpt4-1_prompting_guide?featured_on=talkpython" target="_blank" >cookbook.openai.com</a><br/> <strong>Watch this episode on YouTube</strong>: <a href="https://www.youtube.com/watch?v=0an8FbTfZNE" target="_blank" >youtube.com</a><br/> <strong>Episode transcripts</strong>: <a href="https://talkpython.fm/episodes/transcript/507/agentic-ai-workflows-with-langgraph" target="_blank" >talkpython.fm</a><br/> <br/> <strong>--- Stay in touch with us ---</strong><br/> <strong>Subscribe to Talk Python on YouTube</strong>: <a href="https://talkpython.fm/youtube" target="_blank" >youtube.com</a><br/> <strong>Talk Python on Bluesky</strong>: <a href="https://bsky.app/profile/talkpython.fm" target="_blank" >@talkpython.fm at bsky.app</a><br/> <strong>Talk Python on Mastodon</strong>: <a href="https://fosstodon.org/web/@talkpython" target="_blank" ><i class="fa-brands fa-mastodon"></i>talkpython</a><br/> <strong>Michael on Bluesky</strong>: <a href="https://bsky.app/profile/mkennedy.codes?featured_on=talkpython" target="_blank" >@mkennedy.codes at bsky.app</a><br/> <strong>Michael on Mastodon</strong>: <a href="https://fosstodon.org/web/@mkennedy" target="_blank" ><i class="fa-brands fa-mastodon"></i>mkennedy</a><br/></div>
Python Bytes
#434 Most of OpenAI’s tech stack runs on Python
<strong>Topics covered in this episode:</strong><br> <ul> <li><a href="https://blog.trailofbits.com/2025/05/01/making-pypis-test-suite-81-faster/?featured_on=pythonbytes"><strong>Making PyPI’s test suite 81% faster</strong></a></li> <li><strong><a href="https://x.com/skirano/status/1922651912156897284?featured_on=pythonbytes">People aren’t talking enough about how most of OpenAI’s tech stack runs on Python</a></strong></li> <li><a href="https://www.youtube.com/playlist?list=PL2Uw4_HvXqvb98mQjN0-rYQjdDxJ_hcrs"><strong>PyCon Talks on YouTube</strong></a></li> <li><a href="https://pytutorial.com/optimize-python-import-performance/?featured_on=pythonbytes"><strong>Optimizing Python Import Performance</strong></a></li> <li><strong>Extras</strong></li> <li><strong>Joke</strong></li> </ul><a href='https://www.youtube.com/watch?v=uOoDLOYMdDk' style='font-weight: bold;'data-umami-event="Livestream-Past" data-umami-event-episode="434">Watch on YouTube</a><br> <p><strong>About the show</strong></p> <p>Sponsored by Digital Ocean: <a href="https://pythonbytes.fm/digitalocean-gen-ai"><strong>pythonbytes.fm/digitalocean-gen-ai</strong></a> Use code <strong>DO4BYTES</strong> and get $200 in free credit</p> <p><strong>Connect with the hosts</strong></p> <ul> <li>Michael: <a href="https://fosstodon.org/@mkennedy"><strong>@mkennedy@fosstodon.org</strong></a> <strong>/</strong> <a href="https://bsky.app/profile/mkennedy.codes?featured_on=pythonbytes"><strong>@mkennedy.codes</strong></a> <strong>(bsky)</strong></li> <li>Brian: <a href="https://fosstodon.org/@brianokken"><strong>@brianokken@fosstodon.org</strong></a> <strong>/</strong> <a href="https://bsky.app/profile/brianokken.bsky.social?featured_on=pythonbytes"><strong>@brianokken.bsky.social</strong></a></li> <li>Show: <a href="https://fosstodon.org/@pythonbytes"><strong>@pythonbytes@fosstodon.org</strong></a> <strong>/</strong> <a href="https://bsky.app/profile/pythonbytes.fm"><strong>@pythonbytes.fm</strong></a> <strong>(bsky)</strong></li> </ul> <p>Join us on YouTube at <a href="https://pythonbytes.fm/stream/live"><strong>pythonbytes.fm/live</strong></a> to be part of the audience. Usually <strong>Monday</strong> at 10am PT. Older video versions available there too.</p> <p>Finally, if you want an artisanal, hand-crafted digest of every week of the show notes in email form? Add your name and email to <a href="https://pythonbytes.fm/friends-of-the-show">our friends of the show list</a>, we'll never share it. </p> <p><strong>Brian #1:</strong> <a href="https://blog.trailofbits.com/2025/05/01/making-pypis-test-suite-81-faster/?featured_on=pythonbytes"><strong>Making PyPI’s test suite 81% faster</strong></a></p> <ul> <li>Alexis Challande</li> <li>The PyPI backend is a project called Warehouse</li> <li>It’s tested with pytest, and it’s a large project, thousands of tests.</li> <li>Steps for speedup <ul> <li>Parallelizing test execution with pytest-xdist <ul> <li>67% time reduction</li> <li>--numprocesses=auto allows for using all cores</li> <li>DB isolation - cool example of how to config postgress to give each test worker it’s on db</li> <li>They used pytest-sugar to help with visualization, as xdist defaults to quite terse output</li> </ul></li> <li>Use Python 3.12’s sys.monitoring to speed up coverage instrumentation <ul> <li>53% time reduction</li> <li>Nice example of using COVERAGE_CORE=sysmon</li> </ul></li> <li>Optimize test discovery <ul> <li>Always use testpaths</li> <li>Sped up collection time. 66% reduction (collection was 10% of time)</li> <li>Not a huge savings, but it’s 1 line of config</li> </ul></li> <li>Eliminate unnecessary imports <ul> <li>Use python -X importtime</li> <li>Examine dependencies not used in testing.</li> <li>Their example: ddtrace <ul> <li>A tool they use in production, but it also has a couple pytest plugins included</li> <li>Those plugins caused ddtrace to get imported </li> <li>Using -p:no ddtrace turns off the plugin bits</li> </ul></li> </ul></li> </ul></li> <li>Notes from Brian: <ul> <li>I often get questions about if pytest is useful for large projects.</li> <li>Short answer: Yes!</li> <li>Longer answer: But you’ll probably want to speed it up</li> <li>I need to extend this article with a general purpose “speeding up pytest” post or series. </li> <li>-p:no can also be used to turn off any plugin, even builtin ones. <ul> <li>Examples include <ul> <li>nice to have developer focused pytest plugins that may not be necessary in CI</li> <li>CI reporting plugins that aren’t needed by devs running tests locally</li> </ul></li> </ul></li> </ul></li> </ul> <p><strong>Michael #2:</strong> <a href="https://x.com/skirano/status/1922651912156897284?featured_on=pythonbytes">People aren’t talking enough about how most of OpenAI’s tech stack runs on Python</a></p> <ul> <li>Original article: <a href="https://newsletter.pragmaticengineer.com/p/chatgpt-images?featured_on=pythonbytes">Building, launching, and scaling ChatGPT Images</a></li> <li><strong>Tech stack:</strong> The technology choices behind the product are surprisingly simple; dare I say, pragmatic! <ul> <li><strong>Python:</strong> most of the product’s code is written in this language.</li> <li><a href="https://fastapi.tiangolo.com/?featured_on=pythonbytes"><strong>FastAPI</strong></a><strong>:</strong> the Python framework used for building APIs quickly, using standard Python type hints. As the name suggests, FastAPI’s strength is that it takes less effort to create functional, production-ready APIs to be consumed by other services.</li> <li><strong>C:</strong> for parts of the code that need to be highly optimized, the team uses the lower-level C programming language</li> <li><a href="https://temporal.io/?featured_on=pythonbytes"><strong>Temporal</strong></a>: used for asynchronous workflows and operations inside OpenAI. <em>Temporal is a neat workflow solution that makes multi-step workflows reliable even when individual steps crash, without much effort by developers. It’s particularly useful for longer-running workflows like image generation at scale</em></li> </ul></li> </ul> <p><strong>Michael #3:</strong> <a href="https://www.youtube.com/playlist?list=PL2Uw4_HvXqvb98mQjN0-rYQjdDxJ_hcrs"><strong>PyCon Talks on YouTube</strong></a></p> <ul> <li>Some talks that jumped out to me: <ul> <li><a href="https://www.youtube.com/watch?v=ydVmzg_SJLw&list=PL2Uw4_HvXqvb98mQjN0-rYQjdDxJ_hcrs&index=1&t=8s&pp=iAQB">Keynote by Cory Doctorow</a></li> <li><a href="https://www.youtube.com/watch?v=iURLDirfmno&list=PL2Uw4_HvXqvb98mQjN0-rYQjdDxJ_hcrs&index=6&pp=iAQB">503 days working full-time on FOSS: lessons learned</a></li> <li><a href="https://www.youtube.com/watch?v=o4hyA4hotxw&list=PL2Uw4_HvXqvb98mQjN0-rYQjdDxJ_hcrs&index=21&t=1434s&pp=iAQB">Going From Notebooks to Scalable Systems</a> <ul> <li>And <a href="https://www.youtube.com/watch?v=n2WFfVIqlDw">my Talk Python conversation</a> around it. (edited episode pending)</li> </ul></li> <li><a href="https://www.youtube.com/watch?v=sFAxHLaVKxk&list=PL2Uw4_HvXqvb98mQjN0-rYQjdDxJ_hcrs&index=29&pp=iAQB">Unlearning SQL</a></li> <li><a href="https://www.youtube.com/watch?v=gM2J5plaDmw&list=PL2Uw4_HvXqvb98mQjN0-rYQjdDxJ_hcrs&index=32&pp=iAQB">The Most Bizarre Software Bugs in History </a></li> <li><a href="https://www.youtube.com/watch?v=Cpr0wbopYvU&list=PL2Uw4_HvXqvb98mQjN0-rYQjdDxJ_hcrs&index=36&pp=iAQB">The PyArrow revolution in Pandas</a> <ul> <li>And <a href="https://talkpython.fm/episodes/show/503/the-pyarrow-revolution?featured_on=pythonbytes">my Talk Python episode</a> about it.</li> </ul></li> <li><a href="https://www.youtube.com/watch?v=NE-Oq8I3X_w&list=PL2Uw4_HvXqvb98mQjN0-rYQjdDxJ_hcrs&index=41&t=1322s&pp=iAQB">What they don't tell you about building a JIT compiler for CPython</a> <ul> <li>And <a href="https://www.youtube.com/watch?v=abNY_RcO-BU&t=2s&pp=0gcJCbAJAYcqIYzv">my Talk Python conversation</a> around it (edited episode pending)</li> </ul></li> <li><a href="https://www.youtube.com/watch?v=IhNSINolcSM&list=PL2Uw4_HvXqvb98mQjN0-rYQjdDxJ_hcrs&index=45&pp=iAQB0gcJCY0JAYcqIYzv">Design Pressure: The Invisible Hand That Shapes Your Code </a></li> <li><a href="https://www.youtube.com/watch?v=3-3zy5W2SOw&list=PL2Uw4_HvXqvb98mQjN0-rYQjdDxJ_hcrs&index=55&pp=iAQB">Marimo: A Notebook that</a><a href="https://www.youtube.com/watch?v=3-3zy5W2SOw&list=PL2Uw4_HvXqvb98mQjN0-rYQjdDxJ_hcrs&index=55&pp=iAQB"> </a><a href="https://www.youtube.com/watch?v=3-3zy5W2SOw&list=PL2Uw4_HvXqvb98mQjN0-rYQjdDxJ_hcrs&index=55&pp=iAQB">"Compiles"</a><a href="https://www.youtube.com/watch?v=3-3zy5W2SOw&list=PL2Uw4_HvXqvb98mQjN0-rYQjdDxJ_hcrs&index=55&pp=iAQB"> Python for Reproducibility and Reusability</a> <ul> <li>And <a href="https://talkpython.fm/episodes/show/501/marimo-reactive-notebooks-for-python?featured_on=pythonbytes">my Talk Python episode</a> about it.</li> </ul></li> <li><a href="https://www.youtube.com/watch?v=8utSRblGEB0&list=PL2Uw4_HvXqvb98mQjN0-rYQjdDxJ_hcrs&index=58&pp=iAQB">GPU Programming in Pure Python </a> <ul> <li>And my <a href="https://www.youtube.com/watch?v=57POBhLVbcc&t=2906s">Talk Python conversation</a> around it (edited episode pending)</li> </ul></li> <li><a href="https://www.youtube.com/watch?v=0mZ0AkLimDg&list=PL2Uw4_HvXqvb98mQjN0-rYQjdDxJ_hcrs&index=59&pp=iAQB">Scaling the Mountain: A Framework for Tackling Large-Scale Tech Debt</a></li> </ul></li> </ul> <p><strong>Brian #4:</strong> <a href="https://pytutorial.com/optimize-python-import-performance/?featured_on=pythonbytes"><strong>Optimizing Python Import Performance</strong></a></p> <ul> <li>Mostly pay attention to #'s 1-3</li> <li>This is related to speeding up a test suite, speeding up necessary imports.</li> <li>Finding what’s slow <ul> <li>Use python -X importtime <the reset of the command</li> <li>Ex: python -X importtime ptyest</li> </ul></li> <li>Techniques <ul> <li>Lazy imports <ul> <li>move slow-to-import imports into functions/methods</li> </ul></li> <li>Avoiding circular imports <ul> <li>hopefully you’re doing that already</li> </ul></li> <li>Optimize __init__.py files <ul> <li>Avoid unnecessary imports, heavy computations, complex logic</li> </ul></li> </ul></li> <li>Notes from Brian <ul> <li>Some questions remain open for me <ul> <li>Does module aliasing really help much?</li> </ul></li> <li>This applies to testing in a big way <ul> <li>Test collection imports your test suite, so anything imported at the top level of a file gets imported at test collection time, even if you only are running a subset of tests using filtering like -x or -m or other filter methods.</li> <li>Run -X importtime on test collection.</li> <li>Move slow imports into fixtures, so they get imported when needed, but NOT at collection.</li> </ul></li> </ul></li> <li>See also: <ul> <li><a href="https://docs.python.org/3/using/cmdline.html#cmdoption-X">option</a><a href="https://docs.python.org/3/using/cmdline.html#cmdoption-X"> </a><a href="https://docs.python.org/3/using/cmdline.html#cmdoption-X">-X</a><a href="https://docs.python.org/3/using/cmdline.html#cmdoption-X"> in the standard docs</a></li> <li>Consider using <a href="https://pypi.org/project/import-profile/?featured_on=pythonbytes">import_profile</a></li> </ul></li> </ul> <p><strong>Extras</strong> </p> <p>Brian:</p> <ul> <li><a href="https://hugovk.dev/blog/2025/peps-and-co/?featured_on=pythonbytes">PEPs & Co.</a> <ul> <li>PEP is a ‘backronym”, an acronym where the words it stands for are filled in after the acronym is chosen. Barry Warsaw made this one up.</li> <li>There are a lot of “enhancement proposal” and “improvement proposal” acronyms now from other communities</li> </ul></li> <li><a href="https://pythontest.com?featured_on=pythonbytes">pythontest.com</a> has a new theme <ul> <li>More colorful. Neat search feature</li> <li>Now it’s excruciatingly obvious that I haven’t blogged regularly in a while <ul> <li>I gotta get on that</li> </ul></li> <li>Code highlighting might need tweaked for dark mode</li> </ul></li> </ul> <p>Michael: </p> <ul> <li><a href="https://github.com/git-bug/git-bug?featured_on=pythonbytes">git-bug</a></li> <li><a href="https://www.youtube.com/watch?v=gFiSkcu4kU4&lc=UgxkRBMDgfv8PqbE1iN4AaABAg">Pyrefly follow up</a></li> </ul> <p><strong>Joke:</strong> <a href="https://x.com/PR0GRAMMERHUM0R/status/1637167010365812737?featured_on=pythonbytes">There is hope</a>.</p>
Zato Blog
Enterprise Python: Integrating with Salesforce
Enterprise Python: Integrating with Salesforce
Overview
Salesforce connections are one of the newest additions to Zato 3.2, allowing you to look up and manage Salesforce records and other business data. To showcase it, the article will create a sample Salesforce marketing campaign in a way that does not require the usage of anything else except for basic REST APIs combined with plain Python objects, such as dicts.
If you have not done it already, you can download Zato here.
Basic workflow
The scope of our works will be:
- Creating Salesforce credentials for our integration project
- Defining a Salesforce connection in Zato
- Authoring a service that will map input data to the format that Salesforce expects
- Creating a Zato REST channel that will be invoked through curl during tests
- Testing the integration
Creating Salesforce credentials
To be able to create as connection to Salesforce in the next step, we need a few credentials. There is a full article about how to prepare them and this section is the gist of it.
In runtime, based on this information, Zato will obtain the necessary authentication and authorization tokens itself, which means that you will only focus on the business side of the integrations, not on the low-level aspects of it.
The process of obtaining the credentials needs to be coordinated with an administrator of your organization. To assist in that, the screenshots below explain where to find them.
The credentials are:
- Username and password
- Consumer key
- Consumer secret
The username and password are simply the same credentials that can be used to log in to Salesforce:
Consumer key and secret are properties of a connected app - this is a term that Salesforce uses for API clients that invoke its services. If you are already an experienced Salesforce REST API user, you may know the key and secret under their aliases of "client_id" and "client_secret" - these are the same objects.
Note that when a connected app already exists and you would like to retrieve the key and secret, they will be available under the "View" menu option for the app, not under "Edit" or "Manage".
Defining a Salesforce connection in Zato
With all the credentials in place, we can create a new Salesforce connection in Zato Dashboard, as below.
Authoring an integration service in Python
Above, we created a connection definition that lets Zato obtain session tokens and establish connections to Salesforce. Now, we can create an API service that will make use of such connections.
In the example below, we are using the POST REST method to invoke an endpoint that creates new Salesforce campaigns. In your own integrations, you can invoke any other Salesforce endpoint, using any REST method as needed, by following the same pattern, which is, create a model with input fields, build a Python dict for the request to Salesforce, invoke it and map all the required from the response from Salesforce to that which your own service returns to its own callers.
Note that we use a datamodel-based SimpleIO definition for the service. Among other things, although we are not going to do it here, this would let us offer definitions for this and other services.
# -*- coding: utf-8 -*-
# stdlib
from dataclasses import dataclass
# Zato
from zato.server.service import Model, Service
# ###########################################################################
if 0:
from zato.server.connection.salesforce import SalesforceClient
# ###########################################################################
@dataclass(init=False)
class CreateCampaignRequest(Model):
name: str
segment: str
# ###########################################################################
@dataclass(init=False)
class CreateCampaignResponse(Model):
campaign_id: str
# ###########################################################################
class CreateCampaign(Service):
class SimpleIO:
input = CreateCampaignRequest
output = CreateCampaignResponse
def handle(self):
# This is our input data
input = self.request.input # type: CreateCampaignRequest
# Salesforce REST API endpoint to invoke - note that Zato
# will add a prefix to it containing the API version.
path = '/sobjects/Campaign/'
# Build the request to Salesforce based on what we received
request = {
'Name': input.name,
'Segment__c': input.segment,
}
# .. create a reference to our connection definition ..
salesforce = self.cloud.salesforce['My Salesforce Connection']
# .. obtain a client to Salesforce ..
with salesforce.conn.client() as client: # type: SalesforceClient
# .. create the campaign now ..
sf_response = client.post(path, request)
# .. build our response object ..
response = CreateCampaignResponse()
response.campaign_id = sf_response['id']
# .. and return its ID to our caller.
self.response.payload = response
# ###########################################################################
Creating a REST channel
Note that we assign HTTP Basic Auth credentials to the channel. In this manner, it is possible for clients of this REST channel to authenticate using a method that they are already familiar which simplifies everyone's work - it is Zato that deals with how to authenticate against Salesforce whereas your API clients use the ubiquitous HTTP Basic Auth method.
Testing
The last step is to invoke the newly created channel:
$ curl http://api:password@localhost:17010/api/campaign/create -d '{"name":"Hello", "segment":"123"}'
{"campaign_id":"8901Z3VHXDTebEJWs"}
$
That is everything - you have just integrated with Salesforce and exposed a REST channel for external applications to integrate with!
More resources
➤ Python API integration tutorials
➤ What is an integration platform?
➤ Python Integration platform as a Service (iPaaS)
➤ What is an Enterprise Service Bus (ESB)? What is SOA?
➤ Open-source iPaaS in Python
June 01, 2025
Real Python
Quiz: How Can You Structure Your Python Script?
In this quiz, you’ll test your understanding of the tutorial How Can You Structure Your Python Script?
By working through this quiz, you’ll revisit best practices for organizing your Python scripts, including setting up the main entry point, using imports effectively, and writing code that can be reused as modules or run as standalone scripts.
[ Improve Your Python With 🐍 Python Tricks 💌 – Get a short & sweet Python Trick delivered to your inbox every couple of days. >> Click here to learn more and see examples ]
Zero to Mastery
[May 2025] Python Monthly Newsletter 🐍
66th issue of Andrei Neagoie's must-read monthly Python Newsletter: Free-Threaded Python, Pyrefly vs ty, Big Tech News, and much more. Read the full newsletter to get up-to-date with everything you need to know from last month.
Tryton News
Newsletter June 2025
During the last month we focused on fixing bugs and improving the behaviour of things, speeding-up performance issues - building on the changes from our last release 7.6. We also added some new features which we would like to introduce to you in this newsletter.
For an in depth overview of the Tryton issues please take a look at our issue tracker or see the issues and merge requests filtered by label.
Changes for the User
User Interface
Now we store the Message-Id header from sent emails. This change would later allow to manage the status of bouncing, deferred or delivered.
We now export Many2One
- and Reference
-fields by using their record name. Also we support the export of xxx2Many
-fields as a list of names. The import of One2Many
-fields now supports also a list of names.
New Releases
We released bug fixes for the currently maintained long term support series
7.0 and 6.0, and for the penultimate series 7.6, 7.4 and 7.2.
1 post - 1 participant
May 31, 2025
The Python Coding Stack
What's the Difference Between Zipping and Unzipping Your Jacket? • Unzipping in Python
Today's post is short and visual. You probably used Python's zip()
before. (But if you haven't, you'll figure out what it does in this article, so don't worry!)
But how often have you used Python's unzipping tool?
Eh?! Did you miss this? Do you want to go and have a look for this tool in your IDE or the Python docs? I'll save you the trouble. You won't find it.
But Python does have an unzipping tool, I promise. Confused? Let's start.
Terminology note: As regular readers may have noticed, I use British English in articles on The Stack, with occasional translations to the US variant when needed. In British English, zip is both the noun and the verb—we don't use the word zipper. However, to differentiate between the clothing zip like the one on your jacket and Python's zip()
, I'll use the US English variant zipper to refer to the clothing type.
Zipping • Meet the Team
Meet the team. There are three lists with the names, ages, and locations of four team members in some nondescript team:

Let's visualise these lists:
If you had a choice, you might have chosen to use a single data structure to store these related items since the first number in ages
is the age of the first person in names
, and the first city in locations
is where he's located, and so on. But you don't always have a choice. So, let's assume this is what you have, and you need to work with three separate data structures.
In the Python program, there's no connection between elements from the different lists. The only connection is between elements within each list since they're members of the same list.
But you can zip these three lists together. And this works (almost) in the same way as the zipper on your jacket. One difference is that you can zip as many things as you want. The clothing zipper can only zip two sides of the garment.
Let's add zippers to our lists so you can see this better:
Time to zip up:
If I had more time, I'd figure out a way to create an animation for the next phase. But your imagination will have to do instead. Zip up both sets of zippers:
The zipping action brings elements from different lists together. So, "James"
, 20
, and "London"
are now connected following the call to zip()
. Since zip()
returns an iterator, you can use next()
to confirm that the iterator’s first element contains these three items:
And why not confirm the rest as well?
Each trio of name, age, and location is grouped within a tuple. Let's visualise the new groupings:
If you try to call next()
again, you'll get a StopIteration
exception. Read more about this process in this article if you're interested: The Anatomy of a `for` Loop.
If you're following along in the REPL, you'll now need to recreate the zip
object team_members
since the calls to next()
above exhausted the iterator:
Sorting by age
Now, let's sort the team members by age, from youngest to oldest. You can't simply sort the list ages
since you also want to rearrange the respective names and locations. You can use the zip
object you just created, team_members
:
You sort the tuples yielded by the zip
object team_members
using the built-in sorted()
. You use the function's key
parameter to ensure you sort using the second element (index = 1
) from each tuple. You can read more about sorted()
and the key
parameter, which you also find in other places in Python, here: The Key To The `key` Parameter in Python.
And if you don't like lambda
functions, you can use operator.itemgetter(1)
, which is also more efficient. But that's taking us off course, so let's move on.
Here's the visual representation of the sorted groupings, which are tuples. They’re ordered based on the person’s age:
Do you want to join a forum to discuss Python further with other Pythonistas? Upgrade to a paid subscription here on The Python Coding Stack to get exclusive access to The Python Coding Place's members' forum. More Python. More discussions. More fun.
And you'll also be supporting this publication. I put plenty of time and effort into crafting each article. Your support will help me keep this content coming regularly and, importantly, will help keep it free for everyone.
Unzipping
But, you still want to have separate lists for the names, ages, and locations, as this was the original format of your data.
You started off with three separate data structures, names
, ages
, and locations
. Recall how there was no connection within Python between elements of these different lists.
The zip()
call solved this problem by linking elements from the three lists together. This allowed you to sort them while ensuring that the names and locations swap places along with the ages.
But now you have a connection between a name, an age, and a location, as each tuple contains one of each. However, you no longer have connections between all the names, all the ages, and all the locations.
So, you need to zip the four tuples together! Recall that zip()
can take any number of iterables as arguments. Earlier in this article, you zipped three iterables: the lists names
, ages
, and locations
.
Now, you can zip the four tuples stored in the list sorted_team
. Let's add zippers to these four tuples:
You need to zip these together:
Conceptually, you'd like to write something like this:
zip(
('Sarah', 19, 'Vancouver'),
('James', 20, 'London'),
('Kate', 34, 'Sydney'),
('Bob', 54, 'San Francisco'),
)
You'd need to pass the four tuples as four positional arguments in zip()
. But doing this by hand is too much work. We want to be lazy.
You have a list that contains all these tuples—the list sorted_team
. However, you can't simply pass the list to zip()
since the list is just one iterable, but zip()
needs two or more iterables to zip them together.
Instead, you can unpack the list using the unpacking operator *
. Let's return to our REPL session to complete this:
And here's the visual representation:
You started with the three lists
names
,ages
, andlocations
.You zipped them together to create groupings, each containing a name, an age, and a location.
The zipping enabled you to sort these three-tuples (tuples each containing three items) using the person's age.
Finally, you unzipped the result to get back to having three separate iterables:
names
,ages
, andlocations
. Since these iterables are returned by thezip()
call, they're tuples, not lists. But you can cast them to lists if that's what you want.
Note that the built-in sorted()
returns a list, which you then unpack within the call to zip()
to unzip its contents. You may use other tools in between zipping and unzipping that return iterators or any other iterable. The process remains the same. You'll still need to unpack the final iterable within the call to zip()
using the unpacking operator *
.
Final Words
So, Python does have an unzipping tool, after all. It's the same tool you use to zip up: zip()
. The unzipping process requires the additional step of unpacking the iterable you got from the original zip()
and any further processing performed on the data before unzipping.
And that's exactly what you do with your jacket zipper. You use the same zipper to zip up and unzip. You simply reverse the motion.
Image of zipper used in diagrams by Nina Garman from Pixabay
Code in this article uses Python 3.13
The code images used in this article are created using Snappify. [Affiliate link]
You can also support this publication by making a one-off contribution of any amount you wish.
For more Python resources, you can also visit Real Python—you may even stumble on one of my own articles or courses there!
Also, are you interested in technical writing? You’d like to make your own writing more narrative, more engaging, more memorable? Have a look at Breaking the Rules.
And you can find out more about me at stephengruppetta.com
Further reading related to this article’s topic:
Appendix: Code Blocks
Code Block #1
names = ["James", "Bob", "Kate", "Sarah"]
ages = [20, 54, 34, 19]
locations = ["London", "San Francisco", "Sydney", "Vancouver"]
Code Block #2
team_members = zip(names, ages, locations)
Code Block #3
next(team_members)
# ('James', 20, 'London')
Code Block #4
next(team_members)
# ('Bob', 54, 'San Francisco')
next(team_members)
# ('Kate', 34, 'Sydney')
next(team_members)
# ('Sarah', 19, 'Vancouver')
Code Block #5
team_members = zip(names, ages, locations)
Code Block #6
sorted_team = sorted(team_members, key=lambda grouping: grouping[1])
sorted_team
# [('Sarah', 19, 'Vancouver'), ('James', 20, 'London'),
# ('Kate', 34, 'Sydney'), ('Bob', 54, 'San Francisco')]
Code Block #7
names, ages, locations = zip(*sorted_team)
names
# ('Sarah', 'James', 'Kate', 'Bob')
ages
# (19, 20, 34, 54)
locations
# ('Vancouver', 'London', 'Sydney', 'San Francisco')
For more Python resources, you can also visit Real Python—you may even stumble on one of my own articles or courses there!
Also, are you interested in technical writing? You’d like to make your own writing more narrative, more engaging, more memorable? Have a look at Breaking the Rules.
And you can find out more about me at stephengruppetta.com